
Mark P. Dangelo: In an AI Reimagined Financial World, It Begins with the Consumer (Part 3)
Mark Dangelo currently serves as an independent innovation practitioner and advisor to private equity and VC funded firms. He has led diverse restructurings, MAD and transformations in more than 20 countries advising hundreds of firms ranging from Fortune 50 to startups. He is also Associate Director for Client Engagement with xLabs, adjunct professor with Case Western Reserve University, and author of five books on M&A and transformative innovation.
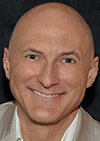
As discussed in Parts 1 and 2 of this series, AI is the current go-to solution set for all organizational and market challenges–regardless of implementation capabilities or an organization’s digital maturity. The promise of implementing advanced, intelligent market and competitive features for many BFSI leaders escalates with each passing week spurred by new industry clouds specifically designed for AI. Compounding the adoption push are Gen-Z and Millennial internal staff enthusiasm to eliminate mundane tasks, uncovering hidden insights and creating greater job satisfaction.
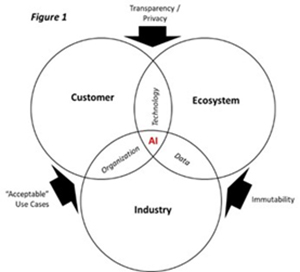
Yet when it comes to the internal and industry digital maturities needed to support the adoption and long-term sustainability of the complex AI implementations, industry strategies to incorporate AI into existing legacy operations represents a potpourri of data robustness, which leads to untold weak points hidden underneath the surface (post-implementation). In general, the promise of AI for BFSI industry groups are stratospheric, but the implications strategy, design and implementation are still being written. As illustrated in Figure 1, successful and sustainable customer-centric AI implementations require many facets–far more than the technology or data.
Moreover, AI strategies, designs and implementation represent a shift of industry mindsets within and across divisional silos and rules-based systems. The designs of the past (and the computing platforms created to mirror them) were based on economies, consumers, regulators and behaviors that are diminished within native digital designs–which AI was expressly created to leverage. Therefore, while the embrace of AI is warranted as part of a quantum shift of computing, it also demands BFSI organizational culture transformations coupled with operating balance and controls that are lagging the technology advancements.
Is the Industry, and Our Organization, Ready to Embrace Data-as-a-Product Monetization?
Today’s automation strategies were designed to streamline paper-based workflows and rules that if we are honest, have diminishing resemblance to the demands of a natively digital BFSI ecosystem empowered by intelligent solutions.
Today, the fundamental and most widespread problem BFSI leaders cite as challenges for AI resolves around data–volume, veracity, validity, variety, value and variability. Yet there is another, more pressing set of challenges–strategizing, designing, and implementing AI using mindsets and methods beyond the traditional rule-based system thinking of prior computing solutions (e.g., PaaS, IaaS, SaaS, BCaaS).
Expanding on the industry value chain of AI introduced in Part 1 of this series, the implications of implementing AI requires not only changes in design-thinking, but also with the strategies of adoption that were created for the explosion of FinTech and RegTech (see Figure below). While the rush to find answers, improve efficiencies, and gain profitability is pushing forward, the underlying success factors reside with three pillars of iterative capabilities–AI strategy, design and implementation.
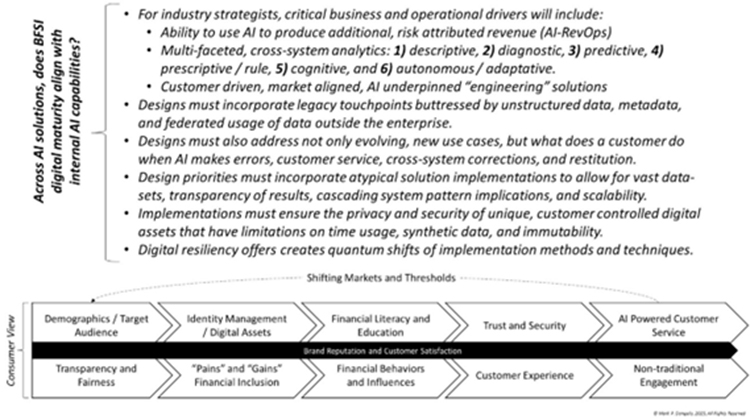
To continually leverage the value chains’ “Shifting Markets and Thresholds” as noted above, BFSI solutions must approach AI customer solutions with two critical cornerstones–adaptation and scale.
How can Industry Build Upon Adaptation and Scale?
To sustain AI industry and organizational solutions where many of the inputs and outputs will cascade between systems, new models of A. strategy, B. design, and C. implementation will be required. As we mentioned, adaptability and scale are foundational horizontal pillars, but each of these will demand alternative vertical slices when viewed within the new AI capabilities. To showcase these framework differences, the following table illustrates the models and their purpose. This framework also consistently applies “Humans in the Loop” involvement which is positively and negatively impacted by capabilities and drivers–it is a give-and take balancing act analogous to a playground “see-saw.”
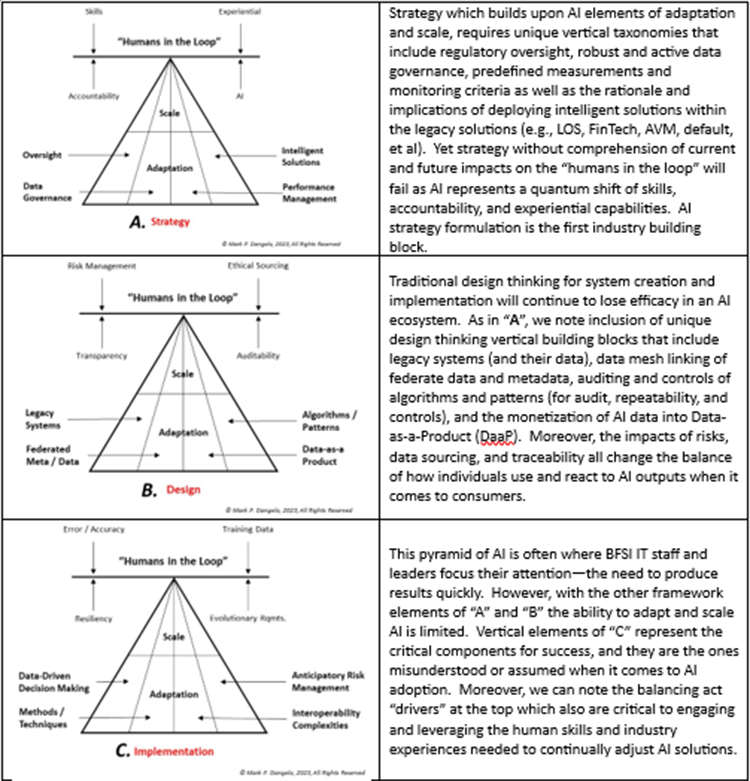
What the above illustrates, is that for industry outcomes to be successful and sustainable the thinking and methods used to craft an innovative solution set is not-trivial–not turnkey (at least not yet). Additionally, when these pyramids of AI demands are linked and viewed iteratively, the cycle of AI improvement showcases why the failure rate for industry AI solutions exceeds 80% and have a lifespan under 15 months.
What is the Model for Cohesively Assembling the Pillars of AI Strategy, Design and Implementation?
As noted, AI is a quantum shift of how computing is executed. It shifts away from rules-driven, system thinking and into ecosystems that are defined by non-structured data to create decisions that learn and adapt. Yet, the mindsets and implementations we use to plan for, architecturally design, and build infrastructure require change. The following model, built upon the items above, shows the vast difference and inherent complexities that move beyond our comprehension of FinTech and RegTech rules-driven system thinking.
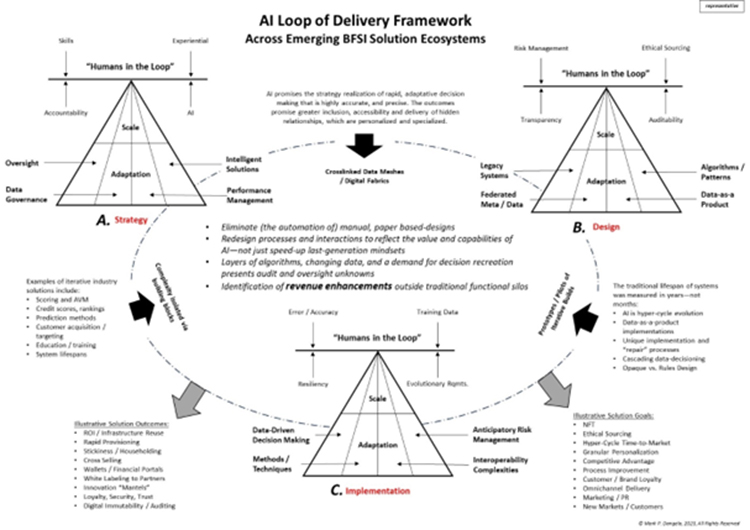
While the above illustration has a number of complex and subtle elements spanning the prior items, what is important to note is that they are part of a loop of AI demands, which unlike rules-driven systems, demands individuals and departments continually iterate AI’s products. Meaning, as these systems evolve, so must the inputs and outputs influencing the goals of rapid-cycle, data-driven decision making.
The integration of AI into organizational cultures accustomed to traditional exchanges is part of the reason AI is undergoing its “halo and horns” attention. Business and IT leaders continue to approach it using the learned lessons of industry tradition–not what is likely and what is already underway.
Why Use a Different Framework?
As we can visually note, the inclusion of AI into industry practices, oversight, and outcomes for BFSI leaders holds great promise–but only if we understand and internalize that sustainable AI is not best suited to traditional strategy and design thinking methods honed during the explosion of FinTech and RegTech these last 15 years.
Echoing this sentiment, Jerome Powell recently stated that the March banking aberrations were a direct result of “the natural human tendency to fight the last war.” That is we view the future using the lessons of history and what made us successful–not necessarily what WILL make us successful in the future. AI for industry, adopting a consumer driven approach, is not only about efficiencies and decisions.
Do industry leaders understand, have mapped, and even deployed “digital twin” capabilities to model what AI needs–will need? Are the mindsets of how rules-driven solutions creating many of the growing pains and discussions we are having around AI and it’s “horns and halos?” In the end, will industry and IT practices have to undergo a quantum shift, much like the embrace of AI outcomes and their impacts on consumers?
As we look forward, Part 4 will closeout this AI series on addressing the digital ecosystems, changing consumer behaviors, and the uncertainty of how AI will perform. However, what is already happening is that AI implementations using traditional rules-driven mindsets will unlikely provide the path to success many BFSI leaders have been staking their brands on achieving–or the long-term prospects of their career.
(Views expressed in this article do not necessarily reflect policy of the Mortgage Bankers Association, nor do they connote an MBA endorsement of a specific company, product or service. MBA NewsLink welcomes your submissions. Inquiries can be sent to Michael Tucker, editor, at mtucker@mba.org, or Anneliese Mahoney, editorial manager, at amahoney@mba.org).