
Mark P. Dangelo: The Digital ‘Butterfly Effect’ — Defining the Consumer and Their Options
Mark P. Dangelo is Chief Innovation Officer with Innovation Expertus, Cleveland, Ohio, responsible for leading and managing global experiential teams for business transformations, digital projects and innovation-based advisory services. He is also president of MPD Organizations LLC and an adjunct professor of graduate studies in innovation and entrepreneurship at John Carroll University. He is the author of five innovation books and numerous articles and a regular contributor to MBA NewsLink. He can be reached at mark@mpdangelo.com or at 440/725-9402.
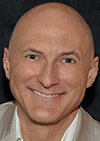
As economic and political impacts shift in 2022, underpinning it all is a silent reality crater exposing audit and systemic risks as digital transformations continuously evolve. For Banking and Financial Services Industries and mortgage leaders, the maturing and iteration of digital solutions crosslinked to third-party data sources represents a cascading and growing liability for every RPA, ML and AI solution.
The chaos and uncertainty of current political actions have cascaded into the business world like inclement weather destroying ecosystems. With the rising tides of innovation ranging from the reformulation of globalization to rising inflation to unfamiliar skill set demands driven by technology, corporate leaders are increasingly consuming data and building ML (machine learning), RPA (robotic process automation) and AI (artificial intelligence) solutions at a frenzied, compounded growth rate of over 65% year over year.
Estimates place these combined markets at over $400 billion by 2025 with between 75% to 85% of financial business leaders stating they are expanding their early RPA / ML / AI usage in 2022 by “x-factors” of investment. However, against these algorithmic models of disruptive innovation, have we (as leaders and IT innovators) reached superior confidence in our data? Have our applications of data governance, curation, sourcing, auditability, ethical, and error management mitigate our myopic focus and attention on the mathematical and relationship formulas rather than concentrate on the quality and clarity of the data oceans and lakes we create in AWS, Azure, Snowflake?
As data ecosystems explode, consumer behaviors transcends and our digital transformations evolve, what happens to investments in these RPA / ML / AI leadership infrastructure initiatives? Like the butterflies flapping their wings in a distant land potentially creating hurricane events thousands of miles away, it can be the simplest of data changes and errors very early in our collections and analysis that cascade across our billions in software and infrastructure investments. These downstream implications increase risks, alienate customers, and bring down regulatory wrath–all because we may be focused on the wrong drivers when it comes to RPA / ML / AI.
Whereas housing markets are defined by location, location, and location, the digital butterfly effect of the consumer and their treatment experience is for the remainder of this decade permanently based in this Fourth Industrial Revolution–and it is all about data, data, and yes, data.
So, Tell Me, What is Your Baseline?
It is overused and accepted axiom that data is the lifeblood for current and future products and services. Yet, countless questions surrounding what, why, and how data is sourced, extracted, manipulated, layered and even compartmentalized are contributing the reality that nearly 90% of prized AI and ML customer solution fail to become operational or last more than 12 months. Why? Is it due to poorly defined algorithms? Were the pilots fatally flawed? Was it a lack of skills? Did the outcomes produce errors or did we use the wrong use cases? Did the consumers reject the channel impacts and outputs produced? Was it something else?
Another and rising realization of our advancements in data technologies and sciences are that data itself, its volume, velocity, variability, variety, value, and veracity (i.e., the Six-V’s), are creating and then quickly terminating dependencies across models. Subsequentially, the codification of business-as-usual data supply chains are in constant flux rendering invalid the codified and decision-based cause-effect relationships and risks once gleaned from the Six-V’s of digital sources.
It is now apparent that as data explodes, the downstream impacts of how these fields and values are utilized plays an out-sized return on the products and services customized for the consumer. That is data continual adjustments, however small, are materially impacting cascading interpretations of data sources thereby impacting targeted demographics, profitability and regulatory compliance. The “butterfly effect” for consumer data is growing as the Six-V’s expand. Ask yourself, what is more important to Google–the data or the downstream implications of the original individual elements that they can sell to advertisers and research organizations? Why are individuals increasingly adopting and deploying software that stymies Googles outputs based on consumers feeding garbage into their prying collection systems?
We know that most of the data we collectively use across financial services is sourced outside of the corporate data centers and cloud providers. Today in BFSI (Banking and Financial Services Industries), just under 40% of data is directly sourced from consumers as part of the banking products and services being offered to them. This means that the 60% of data utilized to target and rate consumers is fed from outside the enterprise by the explosion of the Six-V’s which is then factored into our decisioning and analytical software from outside of our systems-of-record. Therefore, when we ask, “Do we own the customer relationship?” it truly depends on the data sources used to make the downstream recommendations.
Continuous Digital Transformation Maturity
From a systemic risk standpoint, do we understand how small variations or even errors (with the control limit norms) introduced in our data warehouse expansions and the ETL (extraction, transformation, and load) / LTE (ETL in reverse) / API (application programming interface) feeds create material changes in RPA / ML / AI outcomes?
New research and predictions put forward in the ScaleUp:AI conference in April 2022 by AI-pioneer Andrew Ng reinforces the reality that to sustain RPA / ML /AI in a large-scale production setting a course correction of approach and importance is needed–it is the data, not the models where improvements should be concentrated. Fitting models to ever changing and expanding data is, per the session synopsis, a critical driver of ROI RPA / ML / AI failing in over 85% of the solutions introduced. This data first against a continually changing model base is opposite to many current efforts where the data is used reinforce and expand the algorithms. Perhaps we should reconsider the $100K+ paid for entry level data scientists?
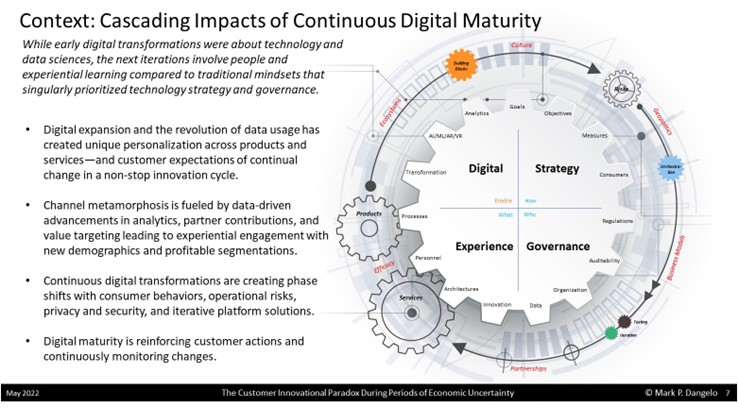
What is forcing this new, agile approach to RPA / ML / AI? We likely have no farther to look than our corporate embrace of digital adoption and transformation and the digital realities and maturities it has introduced across the enterprise and partner relationships.
As noted in the graphic, the components of strategy, governance, experience, and digital are continually changing resulting in “cogs” across the RPA / ML / AI solutions impacted by actions and events some of which are external. The Six-V’s of data coupled with co-dependent factors influence the products and services not in years–but in weeks and months.
The data impacts across the digital supply chains are upending axioms for total cost of ownership (TCO) as well as ROI (return on investment) and competitive distinctions. When overlayed with the current management focus on analytics and key performance indicators (KPI’s), the delivery cycle times are materially elongated relative to the advancements in data, innovations and consumer behaviors. Translation, we cannot iterate analytics fast enough to meet digital innovation usage and downstream linkages.
In the end, it is precisely our experiences with digital transformation which has comprehensively altered how projects, programs and vendor relationships are managed when it comes to RPA / ML / AI. This in turn begs the obvious question: “What should we adopt to be able to leverage this shift of focus?” How will these changes benefit consumers, our products and our time-to-market?
A Beautiful Name for a Highly Complex Beast
If we take seriously the projections of economic flattening or recession, lasting inflationary pressures and a consumer and worker shift where macro ecosystem actions will have a pronounced impact on credit and secondary markets, what can we do to prepare for these impacts against a realization that digital expansion will be as prevalent as billionaires heading into space?
To become adept at the innovation shift and governance demands, business and technology leaders will need to become highly skilled at compartmentalization, creating building blocks, deploying solutions in layers using iteration techniques, and wrap it up with a silent leadership skill of orchestration.
In our software-as-a-service (SaaS) and increasingly no-code / low-code (NC/LC) solutions, the ability to compartmentalize functions and discrete capabilities is exceedingly important. With complexity and advancements in augmented reality (AR) and virtual reality (VR) seeking to be linked to physical offerings (i.e., Metaverse), consumers are demanding product and services experiences—not just transactional fulfilment. To react and adapt in the timeframes of rapid innovation, these compartments need to be cognitively defined as building blocks (e.g., think Legos). With functions defined and placed inside a black box container, organizations can assemble and disassemble product and service offerings and deliver a regulatory compliance audit trail that is both repeatable and amorphic (i.e., data isolation modules, DIM’s).
When organizations assemble these compartmentalized, building blocks into new arrangements or sequences, the integrity and data validity remain, and the consumer is allowed to customize their offering–and organizations can be confident that there is limited to no bias in their recommendations. If the assembly is intuitive, then what management leaders must adopt is orchestration–across vendor products, across internally constructed silos, and across partner platforms. Like the conductor of an orchestra, leaders can alter who is in “first-chair,” who is in “second-chair” based upon consumer needs and their changing behaviors. Moreover, the rapid response and digital transformation audit adjustments (see thought capital from Deloitte and Accenture), allows for expanded roles and greater skill leverage when compared to traditional software deployment.
In conclusion, digital transformation is not an end state. Additionally, digital transformation and the ownership of the data is not steady state. And no, most enterprise leaders have not come to grips with these comprehensive adjustments and iterative deployment designs. As an example, and what is fueling a superheated corner of the MAD (merger, acquisition, and divesture) events, are those leadership firms seeking to “account” for the data they utilize. For like the butterfly effect on the downstream process actions, it is sometimes the smallest change of data that can have outsized impacts on profits and viability. The maturing of digital transformation has made the management of these data butterfly effects real and growing with our digital and cyber defined consumer lives.
With economic conditions now in flux, what strategies are you prepared to advance to mitigate the risks? Who owns the consumer and their data? In a world of digital, how will you compete, retain and differentiate your offerings? The maturing of digital transformation has brought full circle the reality that the Six-V’s of data is once again the starting point for consumer solutions–the cycle per the graphic model presented above is repeatable, iterative and continuous. The false axiom for BFSI leaders who leveraged transaction solutions for competitive distinction ended when industries fully embraced digital transformation back in 2018.
So, tell me, can you account for the data across the entire digital supply chain impacting RPA / ML / AI beyond the initial pilots? Tell me, how do you assemble and disassemble the feeds and algorithms that act on data when data is two to four times greater every 18 to 24 months? Tell me, what do you expect your budgets to become when RPA / ML / AI solutions are legacy–now measured in time spans of months, not years? Tell me, what do you think the regulators will say when they “audit” your RPA / ML / AI offerings to consumers–and you really can’t explain why they didn’t evolve with the data?
The digital butterfly effect has arrived–and what will you do to adjust when the markets are not generating cash and profitability as they have since 2011? Sometimes it is the smallest of inputs which can create the largest impacts all a consequence of digital transformation maturity.
(Views expressed in this article do not necessarily reflect policy of the Mortgage Bankers Association, nor do they connote an MBA endorsement of a specific company, product or service. MBA NewsLink welcomes your submissions. Inquiries can be sent to Mike Sorohan, editor, at msorohan@mba.org; or Michael Tucker, editorial manager, at mtucker@mba.org)