
Mark Dangelo: A House Built on Data Sands
(This article is also available in an AI-generated storytelling format.)
AI is exposing the troubling state of data within industries. As a result, data modernization initiatives are critical imperatives, which can no longer be ignored or bundled with one-off AI solutions modeled from the technical debt of traditional systems design.
There are numerous reasons AI is evolving from a “savior” to an “uncertainty.” That may seem a fallacy given the last three years, but as the capabilities, complexities, layers, and segmentations rapidly evolve, it has ushered in vast innovative technologies, demands for data, and process transformations. Factor in that every second industry adds 4,700 TB of data (i.e., over 1.2 billion songs) exceeding $1 trillion in (data) value in just five years.
Additionally, AI scope and transitory lifecycles are misaligned with traditional implementation approaches. The best analogy might be laying the magnetic tracks in front of a speeding bullet train moving at 400 MPH. The “hype cycles” are running directly into industry domain realities, which are uncovering new risks, regulations, challenges, and politics—it is an emerging perfect storm of continuous, intelligent innovation.
Yet, achieving balance between the benefits and chaos of AI is the new reality for all future systems, processes, and transformations. AI capabilities are growing, becoming segmented or layered, and utilizing discrete AI agents all seeking to mitigate the 20-year legacy system debt continually inhibiting next-gen adaptability. What will the cost be when traditional systems and design approaches fail to meet the demands of iterative AI solutions?
AI expectations for industry leaders is uncharacteristically high, given the last two years of per loan profitability as 2025 is forecast to bounce over 25% off of 30 year volume lows—yet the industry is also at risk of repeating prior mistakes (see Déjà vu: AI is amplifying the mistakes of the past).
AI Built Upon the Data Architectures
For mortgage and banking, financial services and insurance (BFSI) leaders, these exponential introductions of endless AI advancements and abilities continue to strain the organization, its customers, and its partners. Additionally, the exploding burden of adoption is hindered by existing budgets, skill sets, operating procedures, regulations, security, privacy, and most importantly, useful data to feed the models, identify event-driven extensions, and ensure that models and algorithms are adaptable. When combined with ever changing innovation and solution approaches, the transitory demands of AI create risk, uncertainty, and chaos.
Whereas the importance of swelling AI capabilities highlights process and profitability potentials, data aggregation, quality, and auditability continue to be simultaneously a primary challenge and opportunity. Standards (e.g., MISMO) represented the starting point for AI model inclusion, but as data becomes federated, cascading, and feeding multiple systems, language models (both small—SLM and large—LLM), RAG, et al, standardization was just the beginning. Standards are the entry fee to participate in industry not the endpoint for AI, event-driven data demands.
For leadership to secure the greatest return for their AI investments, organizations must address the comprehensive design of data within and across the silos of systems. While data stacks have previously been discussed here at the MBA (see, Data Stacks—a 25 Year Journey that Began with MISMO), it is the logical organization of the data, its integration, and its sourcing that utilizes the data stacks to achieve reusable and adaptable data sources. How will organizations integrate and govern data across a mosaic of data foundations?
While the architecture of data stacks seems esoteric without precise tools mentioned (e.g., Apache, MuleSoft, AWS, Google, Databricks, Informatica, et al), tools represent across rapidly adapting AI solutions the “technical debt” that increasingly hinder intelligent system adoption and continuous adaptation. To move beyond toolset or vendor software axioms within IT departments, AI leaders (e.g., CAIO, Chief AI Officer) are utilizing new data architectures with data stacks to break requirements into manageable, measurable projects interconnected and deterministic.
Given the rapid cycle times of AI applications and agents, the traditional approaches of selecting toolsets to define the data architecture will be a mistake. Moreover, the sunk costs with existing system and data management software should not be prioritized over the requirements necessary for emerging AI orchestration that may sunset numerous industry tools and approaches.
The Missing Piece—Data Weaves
Data design, ingestion, and linkages are critical for BFSI and mortgage solutions and frequently linked to their traditional systems design implementations. To move beyond these domain centric designs, the expansion of the data architecture is orchestrated around three distinct categories—data mesh, data fabric, and most recently, data weaves. Each grouping, as defined by discrete tools and addressing logical parts of robust data stacks, delivers business and technology AI demands.
Below is a high-level representation of the differences between each and how these categorizations can be generated for new and expanding AI enabled use cases utilizing a common data design.
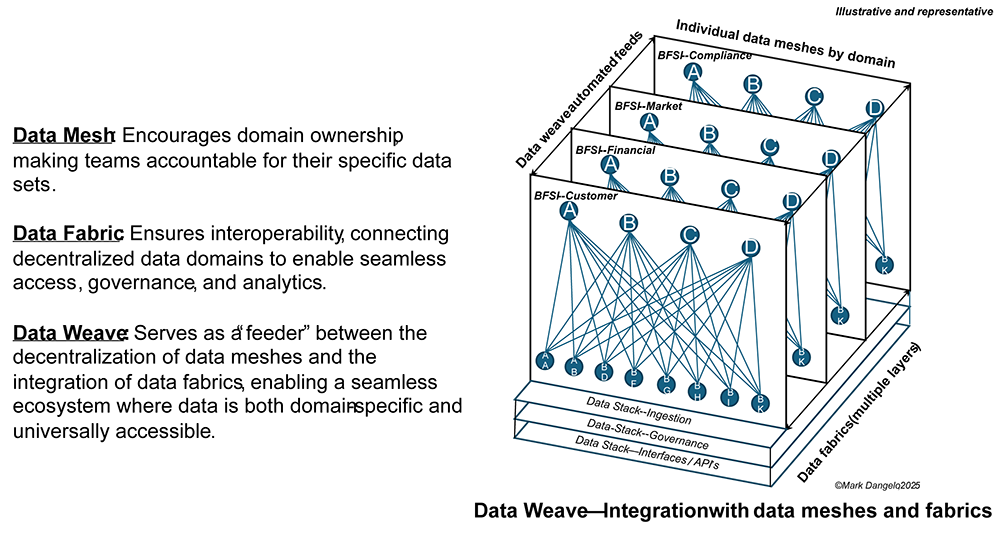
The above illustration shows the segmentation, the orchestration that is needed for AI to adapt. AI is a data-driven, event defined set of interconnected solutions ingesting increasingly diverse data sources. While solutions like Gen-AI (Generative AI) began using large language models (LLM’s) containing billions upon billions of data parameters, new AI solutions are now “fine-tuning” these training principles using smaller datasets often from within the enterprise and across its data domains.
Meaning, to ensure that the organization does not invest in an AI capability that cannot scale, that will not adapt, and that represents a short-term, one-off expense, it all begins with the data. As AI grows more complex, it will be the segmentation of the data that endures. To understand how, we will define the characteristics of the three primary logical structures introduced above.
Characteristics | Domain Integrations (Data Meshes) | Cross-Domains (Data Fabrics) | Data Ingestions (Data Weaves) |
Focus | Domain-specific Data architecture Collaboration and orchestration | Automated integrations Intelligent data management Enterprise | Integration Transformation of dataflows |
Data Ownership | Decentralized Domain owners | Centralized Abstract roles and profiles | Centralized Managed by middleware |
Governance | Federated Shared policies | Automated metadata management | Centralized governance of data flows |
Adaptability | Tailored to data domains Driven by owner security and privacy | Multimodal data environments Unification layers | High for integrating diverse sources |
Interoperability | Interconnected at the domain level | Abstractions / logical overlays Connects multiple ecosystems | API’s Connecting pipelines ETL |
Integration | Shared datasets across domains Manual across-domains | Unified data access across environments Extensive AI / ML | Orchestration Scheduled jobs Dynamic mapping |
Scalability | Scales within domain growth | Enterprise wide | Limited to specific workflows |
Complexity Management | Requires domain alignment Medium to high | High—requires cross-domain resolutions and mapping | Low to medium across data streams |
Data Democratization | Moderate to high | High | Low to moderate |
AI / ML Design | Direct integration at the domain level | Centralized, reusable integration | Indirect requiring tools and automation |
Architecture | Large, decentralized organizations | Large, complex Hybrid or multi-cloud | Heavy integration tasks with defined I/O |
Outcomes | Foster data democratization | Realtime data insights | API transformations ETL / ELT pipelines Connecting legacy systems |
As we navigate a rapidly evolving mortgage landscape, one truth becomes increasingly clear—the ability to innovate hinges on the robustness and evolution of internal and partner data. The cost of not holistically addressing the data fuel needed for AI capabilities cannot be underestimated—provisioning, management, traceability, retirement and compliance.
With data being the consistent element for emerging AI capabilities now crosslinked using an integrated architecture, mortgage leaders must accept that traditional data architecture can no longer meet the demands of a world driven by real-time decision-making, personalized customer experiences, and heightened regulatory scrutiny.
In Conclusion
The legacy applications and their encapsulated data were architected as self-contained solutions. Their data foundations did not need to be any more robust than the system and technical requirements as part of the business analysis. This is the technology debt at represents the average age of a BFSI and mortgage system is over 20 years old.
With the enthusiasm of AI and the needs it demands, the data foundations of traditional technical debt now shown to be built upon sand—shifting, limited, and not scalable. With the embrace of data meshes, fabrics, and weaves, progressive operations are moving beyond outdated silos and into a future where data is seamlessly accessible, inherently secure, and tailored to the unique needs of businesses.
These data architectures not only unlock operational efficiency and innovation, but also position organizations to lead in a competitive and compliance-driven environment. The question is no longer whether we adopt these transformative data approaches—but when. For those ready to begin this journey, the time is now to build a resilient, scalable, and intelligent data foundation that will drive the industry’s future.
A watershed moment is fast arriving for the mortgage industry—and it hinges on challenges that have existed for nearly three decades—data. Can the industry make the shift to leverage the growing body and weight of intelligent capabilities that we collectively call AI—or will it cave into the sands of tradition?
(Views expressed in this article do not necessarily reflect policies of the Mortgage Bankers Association, nor do they connote an MBA endorsement of a specific company, product or service. MBA NewsLink welcomes submissions from member firms. Inquiries can be sent to Editor Michael Tucker or Editorial Manager Anneliese Mahoney.)