
Mark Dangelo–Unlocking AI’s Full Self-Learning Potential
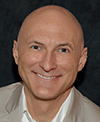
With 11 months to go in 2025, the predictions of mergers, booms, busts, interest rates, and political discourse rise and fall daily. The rutters that once guided the mortgage banking industry after cratering into a 30-year low watermark are being called into question—and vigorously debated. However, the industry is also experiencing unprecedented shockwaves from emerging technologies all bundled under the label of artificial intelligence.
Global strategy firms McKinsey, BCG, and Bain highlight the trillions in value of AI technologies in shaping future economic endeavors, but they are also quick to point out the demands of application context, organizational change, cascading inputs and outputs, and of course, widescale process redesigns against a backdrop of rapid-cycle advancements.
Yet, there is an AI foundational segment that is often addressed with a “Jedi wave of the hand”–data. It is data that feeds AI algorithmic models. It is data (produced globally at 4.7 trillion bytes every second and growing compounded at 25+% per year, according to IDC) created by transactional events, and which is used to continually adjust inputs and outputs. And most importantly (and often deficient) is the inclusion of migrated or converted data from legacy systems that are standard’s compliant, but previously unreconciled or included into the continuous training of these increasingly granular and interconnected AI systems.
It is against this background where we find mortgage leaders and their IT infrastructure struggling to adapt to multiple shifts—industry, organizational, technological, and data. While all the shifts are important, it will be data—its foundation, its conversion, its inclusion, and its reuse for all AI systems—where this article will concentrate.
Rationale for Data Continuous Demands: Conversions, Migrations, Events
Why has data inclusion from prior systems or outside third parties (e.g., data aggregators coupled with ethical sourcing) become critical to the continuous improvement and viability of AI systems? How do data standards impact conversion and event migration (e.g., rules-based versus principle-based conversion)? Additional data requirements complexities impact AI solution scalability, performance, recovery, self-learning, legality, compliance, and most importantly, accuracy of the models and their on-going adaptation.
In today’s mortgage landscape, data has become the foundation of competitive advantage. From delivering personalized customer experiences to ensuring compliance with complex regulatory frameworks, the ability to harness data effectively is no longer optional, it’s a mandate for survival and growth. However, as data ecosystems (i.e., multidimensional, multimodal) become more intricate and complex, organizations are grappling with challenges of transparency, auditability, recovery, and interoperability. These challenges are not just technical, they hinder strategic opportunities, erode customer trust, and slow down the adoption of intelligent technologies like AI.
To fully leverage the potential of AI and intelligent applications, mortgage and bank, financial services and insurance (BFSI) leaders must reimagine their data strategies. This requires: 1) embedding transparency to ensure every data point is traceable and trustworthy, 2) implementing auditability to meet stringent compliance standards, 3) building recovery mechanisms to safeguard against disruptions, and 4) fostering interoperability to enable seamless data exchange across platforms and ecosystems.
Together, these elements create the foundation for a resilient, agile, and innovative data-driven organization.
Armed with the rationale for “why” data inclusion (e.g., industry, organizational, cross-platform) are requirements, the “how” represents the call-to-action (CTA) that organizations in their thirst for AI adoption, fail to adequately address. So, what would a framework of inclusion look like for mortgage firms and their siloed vendor solutions?
Implication: A Foundation Framework for Inclusion
As organizations continue their digital transformation journeys, the need for robust, scalable, and consistent data architecture has become paramount. A multi-dimensional data architecture, including the data inclusion segments, provides the framework for analyzing complex relationships across diverse, cross-process datasets. From financial reporting to real-time analytics, the power of these architectures, and how they include data instances, fuels the ability to unlock insights that drive strategic decisions across all emerging AI solutions.
If a critical data requirement (e.g., MoSCoW systems design prioritizations) is to deliver reusable, accurate, and adaptable data for layers of AI solutions, then organizations can use the categories in Figure 1 to frame a high-level approach. Whereas a number of these classifications look traditional, we have as part of the adaptative process AI within it to assist with data movements, layering, synthetic creation, and even governance. It is a next-gen design that leverages forward thinking data conversion software (e.g., Gladstone Computer Services) with scalable storage, master data management (MDM) and governance software (e.g., Snowflake, Databricks, Informatica, etc.).

However, to achieve value from Figure 1, additional details and design criteria must be added to ensure requirements and priority management for a continuous improvement inclusion architecture will adapt to the capabilities of AI software, hardware, and languages. Figure 2 represents the next step in moving from idea into design.
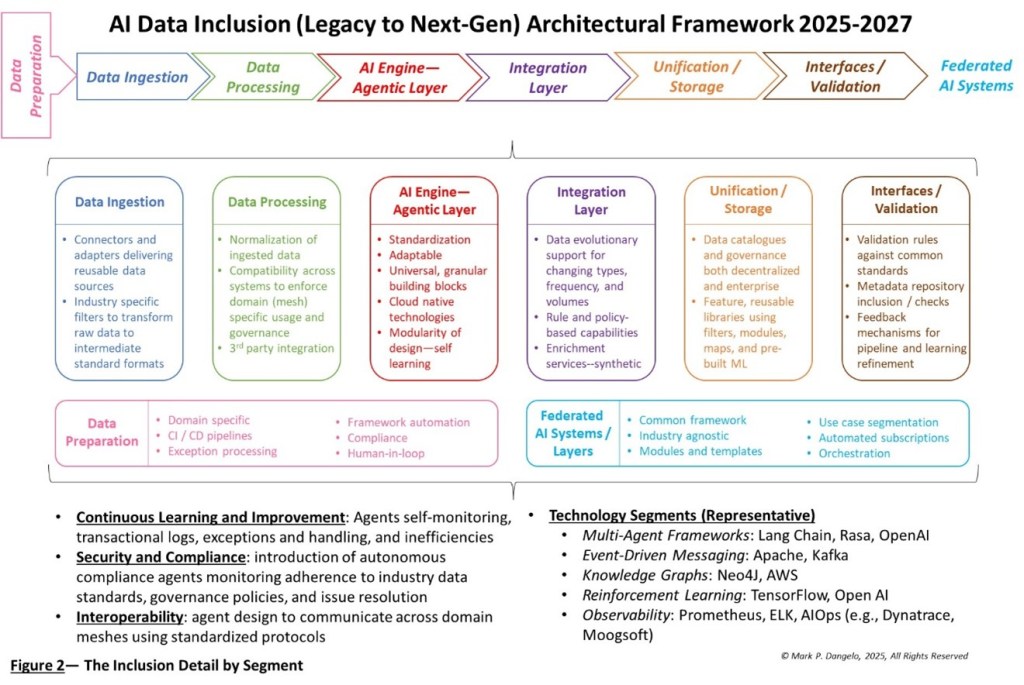
Figure 2 represents the requirements necessary to deliver audited event, transactional, and archival data for intelligent systems that demand source lineage and accuracy. If every new AI system relied on its own data independent of the other intelligent solutions, then which source of data is correct? Is it auditable and traceable? What happens if each data source is independent as self-learning AI systems alter their outputs based on the current data inputs?
For some, it may appear that we are having an academic discussion on the use of data rather than a business-defined, operational one. However, if we look at the history of mortgage and BFSI software in the last 15 years, the rise and inclusion of FinTech and RegTech solutions helped propel advanced solutions even as many of them fragmented the data into storage silos for their own capabilities, reporting, and manipulation. This is created “lock ins” and costly ETLs and APIs to free the data from its functional or original solution—that is it demanded new interoperable requirements to make data available for reuse across the enterprise.
Now if we use history to develop our data architecture of the future, a) will we even be able to unlock data from discrete AI solutions given their adaptation or self-learning, b) will we be able to trace data to the source, and c) how will it be trusted across hundreds if not thousands of cloud AI solutions for each enterprise?
Data Inclusion: Enabler of AI Autonomy, Auditability, and Adaptability
AI’s alphabet soup of acronyms is as impressive as it is confusing. Every week, new terminology, segments, and features are introduced with great fanfare (e.g., LinkedIn Infographics and videos). Moreover, with the vocabulary and system ideations of AI solutions increasingly stacked upon one another, there is a bewildering array of options, designs, and outcomes. What “new features” will survive? Will it be scalable? Do we have the skills to implement, adjust, and integrate? And how is all the data brought together? For industry executives and leaders, it is nothing short of (scary) impressive given the short three-year incubation of applications, skills, and advancements.
Breaking apart Figure 2 and the requirements within into a framework of capabilities and offerings, we see that Figure 3 adds another layer of “flesh” to the inclusion and migration design. Also of note is that on the outside of the data migration “wheel” is the inclusion of advanced data segmentation designs—data meshes, data fabrics, and data weaves.
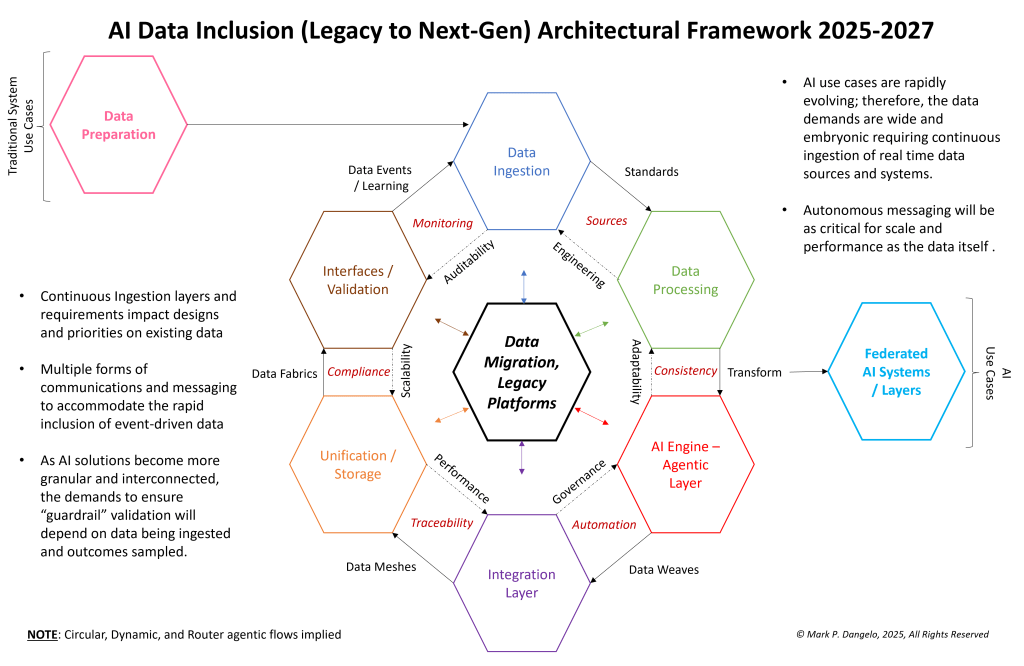
For an industry unaccustomed to cross-vendor data integrations (i.e., non ETL, API), the above approach focuses on data inclusion, which creates a reusable and extensible design that delivers data—not just systems encapsulating data. Moreover, as AI becomes more granular, refined, and interoperable (e.g., high-volume messaging—ISO 20022, AI agents), the focus on data first, systems second cannot be achieved using the system ideations and development approaches of tradition.
To put a finer point on why data inclusion beyond the initial AI training set is so important for the longevity and accuracy of growing network array of discrete industry AI solutions can be found within the speed advancements and ingestion rates of AI designed hardware. In January 2025, Nvidia’s latest AI chip can perform mathematical computations in one second what would take a human 125 million years performing a calculation every second. While mind-boggling, it showcases the data demands, ingestion commonalities, and architectural priorities that feed the rising capabilities of all AI solutions, not just the one that is being piloted.
In Conclusion
Data and its continuous inclusion into a growing array of interconnected AI solutions demands the “breaking down of (vendor) silos” that have dominated the industry. The data architecture to feed intelligent systems are unique to each organization, but based on common standards (e.g., MISMO, ISO 20022, models, and technologies) which are consistent.
In many areas encompassing systems, processes, and designs, AI has permanently shifted operations, IT, and compliance discussions. AI has altered skill priorities, cultural compositions, decision making, and or course, (technology) tool usage.
What we have discussed is how data ingestion is now a critical, continuous core competency for nascent AI infrastructures (beyond GPT / NLP LLM usage). Adopting data transparency, auditability, recovery, and interoperability unlocks the full potential of your data assets, ensuring they are not just operationally robust, but also AI-ready. By aligning your data strategies with architectural ingestion principles, organizations will not only meet today’s regulatory and operational demands, but also future-proof the enterprise for tomorrow’s layered, intelligent, data-driven opportunities.
As industry sectors increasingly turn to multiple forms of AI for competitive efficiencies, one challenge remains universal: ensuring the right data is in the right format to power these systems effectively. It is the misapplication of this goal that contributes to the alarming failure rate of over 85% for production AI systems (Forbes) months after full enterprise deployment.
Legacy systems often contain invaluable structured and unstructured data, but without proper conversion and preparation this data can become a bottleneck rather than an enabler. By focusing on legacy and event data inclusion as a foundational cornerstone, you can unlock the full potential of your AI initiatives.
Investing in data inclusion isn’t just about cleaning up old systems; it’s about creating a common foundation that future-proofs your organization’s AI capabilities and delivers consistent, auditable, and measurable business outcomes. For a mortgage industry hoping for better days, it begins with the data—its inclusion framework above—to keep these AI wonders of technology viable beyond their initial introduction.
A question no one is asking if AI systems fail or are replaced, how do you migrate application specific data that you really cannot trace, lacks balances and controls, and fails the most basic of regulatory and audit guidance? In an AI (to AI) world, it all begins with the data.
In the end, no amount of volume, interest rates, housing supply, or mergers and acquisitions (M&A) will fix fundamental or fatal data provisioning. With AI solutions, we just reach the end much faster and with opaque understanding.
(Views expressed in this article do not necessarily reflect policies of the Mortgage Bankers Association, nor do they connote an MBA endorsement of a specific company, product or service. MBA NewsLink welcomes submissions from member firms. Inquiries can be sent to Editor Michael Tucker or Editorial Manager Anneliese Mahoney.)