
MBA Premier Member Editorial: AI in SaaS: Build It, Borrow It, or Just Wrap GPT?
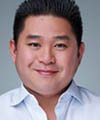
Tim Nguyen is CEO & Co-founder of BeSmartee
Across the mortgage industry and beyond, the push to integrate artificial intelligence is accelerating. Whether you’re a technology vendor, leading an internal product and engineering team or are a buyer of AI tools, the question is no longer whether to use AI — it’s how to use it effectively.
In this environment, three common approaches are emerging:
• Use a hosted large language model (LLM) like OpenAI to launch features quickly
• Build your own AI stack in-house for full control and long-term differentiation
• License the foundational AI infrastructure like AskBobAI to accelerate speed-to-market without losing flexibility
All three approaches have merit, depending on your organization’s goals, capabilities and appetite for investment. However, the differences in cost, time and risk between them are significant. Read on to understand the pros and cons of those three approaches.
The Case for Wrapping OpenAI
Most organizations begin their AI journey by leveraging OpenAI (or similar hosted LLM). This is a logical and appropriate starting point. These platforms allow product teams to deliver AI-powered features quickly, without making large upfront investments in infrastructure or machine learning expertise. Engineers can ship prototypes within weeks, enabling the business to gather feedback, test value hypotheses and demonstrate progress.
In the short term, this strategy is compelling. For many teams, it enables valuable capabilities such as summarizing loan disclosures, answering borrower questions or analyzing underwriting exceptions with minimal friction.
However, relying exclusively on OpenAI (or other LLM APIs) introduces several long-term limitations:
• You don’t own the intelligence; you’re just a thin layer over someone else’s brain
• Inability to tailor outputs to domain-specific language or logic
• Ongoing variable costs tied to usage and token volume
• Limited differentiation, as any competitor can call the same API
• Challenges with data privacy, traceability and auditability, especially in regulated environments like mortgage
These constraints often lead to the next logical conversation: Building the AI infrastructure internally.
The Blueprint for building Your Own AI Stack
The idea of building an AI stack in-house is appealing, especially for organizations with strong engineering cultures. However, building AI infrastructure is not the same as extending a SaaS platform. It requires specialized skill sets, new tools and a deeper architectural investment than most teams anticipate.
How heavy that lift becomes depends on your starting point. Below are two blueprints: one for organizations building from scratch with a new experienced team, and another for those hoping to leverage their existing engineering resources.
Option 1: Building with a Brand New Team
If you choose to build an AI stack by hiring a dedicated team of experienced AI engineers, ML researchers and data scientists, the upfront investment is high, but the team is focused and they bring the required expertise from day one. This allows for faster execution across each layer of the stack.
Component | Purpose | Time | Cost |
Infrastructure (Cloud, GPUs, MLOps) | Provision and manage compute for AI workloads | 2-4 months | $250K-$1M/year |
Data Ingestion and Extraction | Ingest internal content, clean and extract from structured and unstructured data sources | 4-6 months | $500K-$1M |
Vector Indexing (e.g. FAISS, Pinecone) | Store and retrieve vector embeddings for semantic search | 3-4 months | $200K-$500K |
RAG and Search Orchestration | Implement retrieval-augmented generation pipelines and reranking logic | 4-6 months | $300K-$800K |
LLM Integration and Prompt Engineering | Manage context windows, token optimization and routing logic | 2-3 months | $100K-$500K |
Monitoring and Guardrails | Track usage, detect hallucinations, support human feedback and correction | 3-5 months | $300K-$800K |
Fine-Tuning or Model Training | Fine-tuning or Model Training | 5-9 months | $500K-$2M |
Total Estimated Time: 12-20 months
Total Estimated Cost: $3M-$8M+
This is the fastest and most capable route to owning the full AI stack, but only justifiable if AI is central to your product’s long-term differentiation.
Option 2: Building with Your Existing SaaS Engineering Team
Many organizations look to accelerate development by repurposing their current engineering team to build AI infrastructure. While this may reduce initial spend, these engineers typically lack direct experience with AI workflows, retrieval techniques or prompt design. They are also balancing competing priorities from the core product roadmap, which introduces delays due to context switching and fragmented focus.
Component | Purpose | Time | Cost |
Infrastructure (Cloud, GPUs, MLOps) | Provision and manage compute for AI workloads | 3-5 months | $100K-$500K/year |
Data Ingestion and Extraction | Ingest internal content, clean and extract from structured and unstructured data sources | 6-9 months | $300K-$800K |
Vector Indexing (e.g. FAISS, Pinecone) | Store and retrieve vector embeddings for semantic search | 4-6 months | $150K-$400K |
RAG and Search Orchestration | Implement retrieval-augmented generation pipelines and reranking logic | 6-9 months | $250K-$600K |
LLM Integration and Prompt Engineering | Manage context windows, token optimization and routing logic | 3-5 months | $50K-$300K |
Monitoring and Guardrails | Track usage, detect hallucinations, support human feedback and correction | 6-8 months | $200K-$500K |
Fine-Tuning or Model Training | Fine-Tuning or Model Training | 6-10 months | $300K-$1M |
Total Estimated Time: 16-26 months
Total Estimated Cost: $2M-$6M+
While this approach may appear more efficient on paper, it often leads to hidden costs: delayed delivery, loss of engineering focus and slower iteration cycles during a time when the AI market is rapidly evolving.
Importantly, these figures do not reflect investments in actual product features. They represent the cost of foundational capabilities, i.e. the “AI plumbing” required before a single business-specific workflow can be built on top.
The Risk of Premature Infrastructure Investment
It is important to recognize that not every company needs to build its own AI stack.
Unless artificial intelligence is the core product itself — rather than an enhancement to an existing platform — the time and capital required to build internally may far exceed the strategic value in the early stages of AI adoption.
This is especially true for vendors in regulated industries like mortgage lending, where trust, transparency and explainability are essential. Building an in-house AI stack that satisfies enterprise-grade requirements for security, auditing and compliance adds an additional layer of complexity that is often underestimated.
So, what’s the alternative?
Licensing the Stack: A Pragmatic Middle Ground
A growing number of companies are choosing a hybrid approach.
Rather than wrapping OpenAI and hoping for differentiation, or attempting to build infrastructure from scratch, these organizations are licensing a purpose-built AI stack that includes the key foundational elements — retrieval, indexing, document ingestion, orchestration and multi-model routing — while retaining full control over the user experience and application logic.
This approach offers the best of both worlds:
• Time to market in weeks, not quarters
• Slightly more costly than OpenAI, but significantly less than building your own AI stack
• No need to divert core engineering talent
• Access to enterprise-grade infrastructure
• Ability to control the interface, data inputs and downstream workflows
• Flexibility to replace or augment components over time
Companies such as AskBobAI offer drop-in infrastructure for companies who need AI to work with internal knowledge — guidelines, overlays, pricing matrices, policy docs — securely and at scale. AskBobAI is not the front-end; they are the foundation. They are not the model; they are the engine that connects it to your data and workflows.
Choosing the Right Path Based on Stage
For teams evaluating their next move, it’s helpful to consider which stage your organization is in and what trade-offs matter most.
Stage | Best Approach |
Exploration and Prototyping | Wrap GPT. Move fast. Test ideas. |
Validated Use Cases and Early Adoption | License the AI infrastructure. Focus your team on the user experience. |
AI is Core to Your Business Model | Consider building, but only after validating demand, value and risk tolerance. |
Conclusion: Build Strategically, Not Reactively
There’s no shortage of urgency around AI today, but urgency should not be mistaken for strategy. While the temptation to “move fast” is understandable, the most successful companies are those who balance speed with scalability and experimentation with a clear path to operational maturity.
For technology teams, the fastest and most strategic path is not to wrap OpenAI and hope for the best, nor to build a complex AI stack from scratch. It’s to license the infrastructure they need, retain control over what matters and stay flexible for the future.
(Views expressed in this article do not necessarily reflect policies of the Mortgage Bankers Association, nor do they connote an MBA endorsement of a specific company, product or service. MBA NewsLink welcomes submissions from member firms. Inquiries can be sent to Editor Michael Tucker or Editorial Manager Anneliese Mahoney.)