
Your Competition is Not Human Anymore
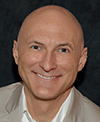
The residential mortgage market for 2025 remains in flux—interest rates, housing supply, consumer sentiment, job security, and economic outlook. And, depending on where you source your news, when you look at the headlines, who you ask within and outside the industry, and political belief and affiliation, the forward-looking outlook can be negative, neutral, or positive. It’s difficult to plan a future when the indicators are interpreted so differently.
However, what is true is that the efficiencies necessary to compete are more elusive than in previous cycles. Cost reductions are made more challenging against the rising demand of technology advancements (e.g., artificial intelligence) limited by corporate technical debt. In addition, productivity and efficiency improvement cycle times take longer to maximize and are shorter as driven by complex operational, fragmented solutions, and most importantly, the rise of data now encapsulated within layers of artificial intelligence (AI).
Markets in Flux—Directions Changing
At the beginning of 2025, the housing and mortgage had strong hopes of a resurgence. Now priorities have shifted and continue to react to policy and market conditions.
• Should organizations maintain their investment course?
• Should leaders change their customer engagement strategy?
• Should technology improvements now be paused or supplemented with more cloud or intelligent capabilities to reduce costs?
• Should a freeze be put into place until at least the second half of 2025 when economic implications become clearer?
• Should we alter our approach to AI provisioning accepting that many of the future competitors no longer “human?”
A final and growing consensus suggests that in times of great uncertainty, leaders need to baseline persistent challenges and eliminate bottlenecks across all processes beginning with the data. In particular, cyclical efficiency improvement programs are repeated every two to four years to drive out new or cascading process variability and encapsulated risks, yet the data is not reviewed or compartmentalized using similar rigor and method criticality. The years 2023 and 2024 witnessed great efficiency efforts due to the lowest volumes in decades, but what is next?
Moreover, traditional management and organizational strategy views digital transformation as a one-and-done initiative rather than as a continuous, iterative series of improvements, which demand rapid-cycle changes especially with the embrace of AI. Since 2023, traditional strategy has been a limiting factor with the arrival of Gen-AI capabilities and its massive data-defined architectures.
Following Gen AI’s embrace in 2H 2023, newer more intelligent AI layers and capabilities have appeared including retrieval augmented generation (RAG) and Agentic AI each more advanced than their predecessor. AI segmentation and advancements continue to explode even with housing and finance sectors being ranked at the lowest levels of adoption.
The Rapid Onset of AI in Uncertain Markets
In research from McKinsey and backed by data from the MBA, the use of data to permanently change processes has resulted in high-efficiency producers being between 30% to 40% superior in cost reductions when compared against enterprises that utilized prescriptive strategy and IT playbooks.
When taken into consideration that the costs per loan originated doubled over the same period (2010-2024), this sustainable savings of over $4,000 per loan provided a lasting competitive advantage and consumer benefit. Was it due to process automation? RPA and intelligent decisioning? Or was it a more foundational series of solutions surrounding reusable data?
AI is a data-driven series of solutions—not a singular approach. With the dozens of new applications added weekly, business leaders and the customers they serve have to navigate layers of intelligent systems—not just prompt engineering or a singular LLM.
These layers, and their cascading (synthetic) inputs and outputs, require a common (enterprise) “digital nervous system” (DNS) spanning current and future capabilities. However, today with the hypercycle introduction of new discrete AI capabilities, the focus is on the functional “appendages” of AI improvements rather than ensuring a common DNS that is utilized by all AI forms.
Showcasing the vast explosion of AI capabilities and to highlight the rapid expansion of now interconnected intelligence, Figure 1 provides the context of components that continue to pressure industry and enterprise not just with adoption—but understanding, integration, and architecture.
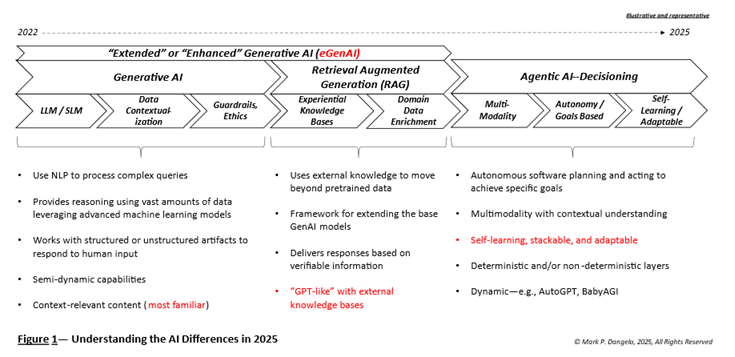
Beyond the technologies, it is the data that links the current and emerging forms of AI into a cohesive whole that must be transparent and auditable. What is necessary for housing and mortgage leaders is to embrace and implement a DNS for AI? How is a DNS architected? Why is the DNS a key success factor (KSF) for future AI implementations?
The DNS Imperative
As Figure 1 illustrates, the progression of everything AI for software and data solutions has the potential to deliver significant opportunities for all functions across housing finance. Yet, the pace of change is also challenging prescriptive methods, implementation techniques, architectural designs, skill sets, and most importantly, data layers and reuse.
The creation of a common DNS is not academic nor is it a one-and done architectural design. DNS represents the foundation that all AI systems are and will be built upon. While the foundation can be extended, modified, or removed, it ensures that whatever the AI functional requirements, the system will not collapse as new use cases and functional capabilities are added.
Using the analogy of a building’s foundation, what are the benefits and rationale for a DNS during times of uncertainty (versus hoping it takes care of itself with more AI introductions)?
• Acceleration of AI solutions: Data reuse (e.g., data-as-a-product, data-as-a-service) across varied use cases and rapidly changing technologies eliminating data silos, fragmentation, and continual rebuilding.
• Data Access and Governance: Standardize access, enforce standards, and ensure consistency across structured and unstructured types.
• Real-time AI automation: Traditional systems lack guaranteed update synchronization and across all AI implementations there are requirements for consistency, accuracy, ingestion, and automation.
• Enterprise-wide AI Integration and Reuse: To ensure AI viability and eliminate continual rework and duplication, the DNS design ensures singularity of purpose across all legacy systems and new AI solutions. Even AI needs a system-of-record.
• Scalability for Growth and Compliance: Traditional approaches concentrate on manual data migrations, integrations, and transformations. With explosive volumes in data, AI systems can alter their outcomes yet the demand for auditability and oversight does not diminish.
Without a common DNS for AI, each uniquely defined solution will have traditional data risks, concurrency, and auditability challenges that historically have plagued legacy software—that is process over data. Table 1 provides the comparison between traditional approaches and those requirements necessary for robust, auditable AI components.
TABLE 1 | Traditional Application Approach | Digital Nervous System (DNS) |
Data Architecture | Siloed data pipelines Rigid integrations | Unified with flexible access Distributed data fabric |
Data Ownership | Centralized IT-driven data controls | Decentralized domain ownership Data mesh specializations |
Scalability | Limited by legacy designs Static models | AI-ready architecture Dynamic scalability |
Interoperability | Complex Point-to-point integrations | API-driven modularity Interoperability across cloud and on-prem |
AI Model Development | Built application by application High duplication | AI-data driven weaves Reusable enterprise models |
Real-time Processing | Batch based Delayed insights | Streaming real-time AI Event-driven architecture |
Compliance and Security | Manual governance High audit complexity | Automated compliance AI-driven anomaly detection |
Expansion Readiness | Difficult integration Cumbersome | Plug and play data ingestion Continuous alignment |
AI for housing finance is only as good as the data that is feeding it—all of it and in all of its forms. Table 1 compares the differences between traditional and AI systems illustrating the business benefits of the DNS involved in revenue growth (e.g., RevOps), faster integrations, and regulatory confidence across all internal and external data assets.
A Modern Approach Driven by AI
In modern AI solutions, DNS requires a foundation incorporating the use of data meshes (vertical domains), data fabrics (cross-domain linkages), and data weaves (continuous data ingestion).
What would happen if your organization could use AI to continuously access changing data instantly, across traditionally incompatible systems? Could your organization and its customers benefit from the leveraged, sustainable efficiencies that an emerging DNS provides?
To take advantage of the DNS with limited resources available, the first question often involves “where to begin?” Forget 5-year data and DNS roadmaps. We’re talking 5-week survival cycles. Evolving, iterative AI solutions aren’t just disrupting industry—they’re rewriting the rules daily. DNS is not an IT initiative—it is a profit driver and a sustainable efficiency enabler.
Step 1: Define business use cases and AI use cases
Step 2: Assess current data against AI requirements
Step 3: Define the data architecture, its layers, and its components (e.g., meshes, fabrics, weaves)
Step 4: Establish a proactive, distributed governance and compliance framework
Step 5: Build a unified data layer designed for all data and AI types
Step 6: Implement an AI lifecycle management model (e.g., iterations)
Step 7: Enable event driven decisioning and automation (i.e., think big, start small)
Step 8: Enable AI decision engines by business unit and domain
Step 9: Continuous assessment and improvement
As many leaders are now embracing, AI isn’t a trend—it’s a tipping point. You either harness it or get overrun by it.
So, what’s the next move? If you want AI to consistently work—not just generate headlines the DNS is the foundation necessary.
In Conclusion
The development of a DNS foundation is not optional. As AI continues to accelerate (see representation in Figure 1), our future collection of intelligent systems will share many of the problems and root causes that are on-going struggles with legacy solutions, FinTech and RegTech.
If we ignore the emerging, foundational requirements now arising across more and more AI capabilities, we will likely find the industry experiencing a déjà vu moment. The uncertainty surrounding markets and consumers, provide a unique opportunity to concentrate efforts on building a solid foundation for current and future AI segments.
AI promises instant decisions, predictive perfection, and the end of inefficiencies, but in reality? Your data is a “hot mess.” Legacy systems choke AI pipelines, bias creeps into models, and broken processes just fail faster—not better. The secret? AI doesn’t replace data strategy—it amplifies its flaws. So, if your foundation is weak, AI won’t fix it, it’ll expose it. DNS is the business enabler for today—and tomorrow.
Finally, as housing and mortgage leaders wrestle with actions in 2025, the horizon for 2026-2027 could finally witness the repurposing of the GSE’s, resurgence of private securitization markets, new financial instruments, and regulatory changes (including property tax treatments) that will ripple across business models and IT systems like a rock being thrown into the middle of a pond. Without a common digital nervous system how can you survive the changes demanded against competition that is no longer singularly human?
The use and implementation of the DNS is now. For institutions thinking they will be able to ignore the mandate of creating an expandable data foundation that spans all their AI systems, 2025 will show the fallacy of that premise. With the uncertainty facing consumers and industry, there has never been a better time to allocate resources to deliver requirements for short-term and long-term scale and viability.
(Views expressed in this article do not necessarily reflect policies of the Mortgage Bankers Association, nor do they connote an MBA endorsement of a specific company, product or service. MBA NewsLink welcomes submissions from member firms. Inquiries can be sent to Editor Michael Tucker or Editorial Manager Anneliese Mahoney.)