
Mark Dangelo: How to Capitalize on The (AI) “Black Swan” Events
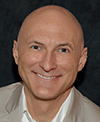
With the glimmer of market movements pushing buyers from the sidelines, regulatory compliance risks will surge in 2025 because of significant advancements across artificial intelligence (AI) solutions. Yet will the explanations of AI features, outcomes, and causalities be sufficient to address regulatory challenges to privacy, security, decisions, and the data foundations underpinning these rapidly changing, opaque innovations?
This article is also available as an AI-generated video here.
Across the mortgage and financial markets there is a story to tell—to understand in the context of what will come to highly regulated segments of the housing markets. Whereas the last two years have been difficult for many participants—especially the consumers—events are in motion that will once again transform traditional practices. From what changes will happen, to who will be responsible, to how they will be accomplished, to why should they be done, the strategic and tactical implementations will not resemble business as usual.
The mortgage industry has had a series of negative events contributing to the bottom line of its members—interest rates, housing supply, policy changes, economic uncertainties, consumer behaviors—all impacting traditional products, services, and technologies. Moreover, demands for digital mortgages, native data-driven outcomes, and the rise of (artificial) intelligent solutions has further exposed traditional system design fragmentation and their cascading data risks throughout origination, servicing, and secondary processes.
Therefore, it should be no surprise that emerging operational demands driven by once-in-a-generation AI black swan technologies have comprehensively shifted traditional application-centric discussions across banking, mortgage, and financial services. Today, the foundations and expectations that are AI are often concentrated around “generative” AI, but this (AI) umbrella also includes many elements—predictive data analytics, neural networks, RPA, cybersecurity, NLP, RegTech, “general AI,” and most recently, Explainable AI (XAI).
To put this into focus, it is NOT that AI by itself is a generational technology, but its ability to comprehensively disrupt the mindsets and models of previous operating systems. Independently taking each of the aforementioned AI umbrella solutions indeed represents an opportunity to improve efficiencies, change the customer experience, and improve competitive differentiation. However, when linked together (cascaded if you will), the comprehensive disruption becomes apparent, yet the methods, techniques, and key measurements are unfamiliar.
Figure 1 provides a representation of four key clusters of events and actions—drivers, markets, call-to-action, and (AI) Black Swan[i] events—that are and will impact the mortgage industry until 2027. The figure begins with the familiar and moves into the unfamiliar with illustrative approaches and categories. Again, taken in isolation from one another, these capabilities look common. When examined across the context of rapid innovation impacting all aspects of the mortgage industry, their linkages represent a generational opportunity for leaders seeking differentiation.
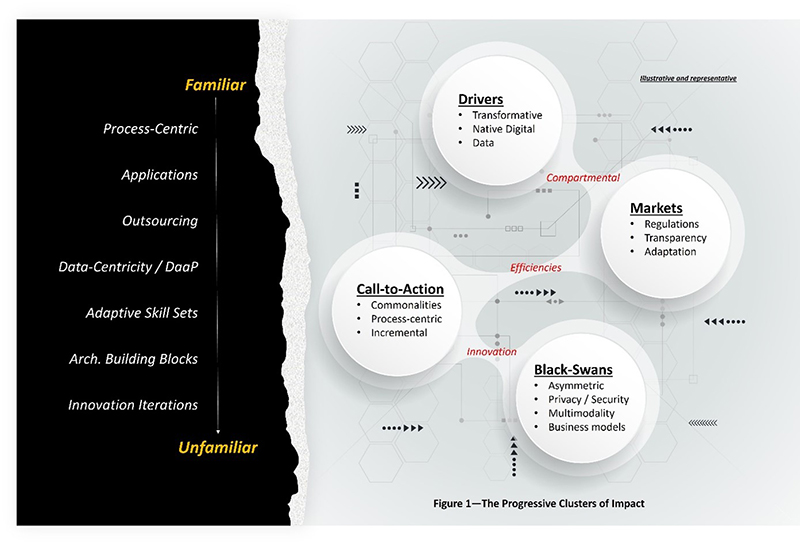
In order to implement real world solutions, let’s unpack a few of the drivers that are influencing the growing chorus of AI use cases within today’s industry and decades of infrastructure built brick-by-brick. This list of drives often includes:
Enhanced cyber and IT security.
End-to-end, digital mortgage solutions (including straight-through processing, or STP)
Smart contracts and blockchain (i.e., standard data immutability)
Predictive data analytics across vast data (i.e., non-internal) sources
Granular customer segmentation and automated response agents
ESG and community impacts
Housing accessibility, fairness, and products
Vast / big data integration (across data marts and lakes) linked to structured data types (underpinned by MISMO standards)
Cloud computing and scalable (on-premises) infrastructures
Personalization and customer engagement solutions
Machine learning for risk management and mitigation
(Adaptive) regulatory compliance and reporting
As organizations and leaders seek greater efficiencies, customer efficacy, and shorter timeframes, it is the last bullet that fuels AI’s advancements—yet tempers the euphoria of AI without “guardrails.” The demands for robust, adaptable regulatory compliance with an industry that minimally includes over 15 different regulatory agencies (i.e., federal and state) is at the heart of every AI solution built on its data stack foundations (see Data Stacks—a 25 Year Journey that Began with MISMO in MBA NewsLink).
If we applied the use cases and touchpoint for just regulatory compliance, we find classifications of systems, processes, legal, and auditability that spans the spectrums or origination, servicing, and secondary markets. These typically include:
Fair-lending and anti-discrimination compliance
Know your customer (KYC) and anti-money laundering (AML)
Document compliance using NLP and Gen-AI
Fraud detection and prevention
Enhanced auditability across siloed systems
Consumer privacy and security
Automated monitoring and reporting
Adaptive, data-driven regulatory technology
If we expand outward, we will uncover several hundred use cases spanning the industry from end to end. It is this complexity and interconnectedness that creates risks for point-based AI solutions—hence why when it comes to scale and longevity, visionary leaders are creating layers or stacks of capabilities.
With the drivers and markets in industry leaders’ sights, the capital and operational investments resulted in “call-to-actions,” which result in corporate (and vendor) initiatives that have representative familiarities against existing systems and implementations. For some mainstream leadership, there is a tendency to accept that if the department or company is executing against one or more of these bulleted items below, that they must be on-track to gain differentiation—that is they are spending money that will yield positive results.
Comprehensive compliance audit against AI strategy and solutions
Common architecture for AI compliance tools and technologies
Building blocks of AI compliance solutions
System failures and operational interdependencies
Enhanced data privacy and security measures
Continuous monitoring and adaptation methodology (e.g., bias and discrimination)
Train and upskill compliance and audit teams
Monitor performance and adjust
Collaborate with industry leaders, oversight agencies and industry peers
Develop / incorporate agile strategies for emerging technologies
However, what is strikingly different from defining and executing these CTA strategies are the implications of their adoption that is unique from the traditional. As noted in prior articles, the failure rates of advanced AI solutions are greater than traditional solutions. WHY?
Is it due to the unfamiliarity of the technologies, data, and algorithms? It is created by a lack of skills needed for implementation and continual maintenance that leads to pilot to production rapid failures? Is it a cultural misalignment that inhibits innovation adoption and continuous improvement? Are their challenges with how these “intelligent” solutions are measured, defined as successful, or assessed as “investment ready?” Perhaps it is a combination of the above or a failure to adapt to hybrid strategies and implementation approaches?
Industry leaders are challenged with many of these questions, yet the singular examination and mitigation of these issues will only marginally ensure success. The ongoing and growing problems reside with the layering demanded of many technologies, numerous personnel, constant transformations, and lifecycle times that are no longer measure in years or by the processes they improve. It is with the layering of these advanced, often AI enhanced and data-driven, where the CTA’s as defined by drivers and markets are no longer sufficient. The following are caveats that will continue to create multiple points of failure for industry leaders (if addressed in isolation):
Cyber and IT security—interconnected, complex, and adaptative
A patchwork of privacy demands spread across state boundaries and special interest groups
Model discrimination and bias risks often increasingly driven by model drift and degradation
Operational changes across complex cloud and on-premise infrastructures
Vendor and third-party risks
Human oversight and skills gaps
Legal and ethical risks
Investment decisions and implementation / scale failures
Reputational risks and market trust failures
Regulatory uncertainty and market shifts
Lack of transparency and explainability (XAI)
When Figure 1 along with the representative breakdowns illustrated above are put into context, a mosaic becomes apparent when charting out the next iteration of housing, finance, and consumer demands. Viewed in isolation, they may not seem like a phase shift or worthy of a black swan label. When viewed holistically, there is a emerging coalescence of designs and technologies that are being defined leading to the conclusion that when assembled we arrive at a generational shift. The last bullet, Explainable AI (XAI) represents another shift surrounding one of the most critical business and regulatory challenges for the next two years—how do you “audit AI?”.
XAI, supported by LIME (Local Interpretable Model-agnostic Explanations) or SHAP (Shapley Additive Explanation) is just emerging, but it is another data point that represents why the mortgage industry will undergo black swan events with the continuous layering of data and AI solutions. Industry leaders hoping to follow their competitors versus blazing their own path may find themselves unable to adjust to drivers, markets, and CTAs without contextualization as illustrated in this article.
AI represents the missing piece that has been promised for forty years as paper-based processes were digitized. Custom software was completely remade with the delivery of SaaS designs. Data was migrated and leveraged using warehouses, lakes, marts, and MISMO. Infrastructure was repurposed and intelligent decisioning became the goal. What was missing was how to put it all together with the speed, scale, and accuracy necessary to make it cost effective. The catalysts were embraced in late 2022, and regardless of industry, nothing will remain the same.
The black swans are coming out of the shadows—who will be the leaders who recognize that these ghostly images are not mirages and fully embrace what is coming? Indeed, as technology accelerates, these black swan events are complexly remaking the who, what, where, why, and how.
[i] The Black Swan Theory describes a surprising event that has a major effect and is often inappropriately rationalized after the fact.
(Views expressed in this article do not necessarily reflect policies of the Mortgage Bankers Association, nor do they connote an MBA endorsement of a specific company, product or service. MBA NewsLink welcomes your submissions. Inquiries can be sent to Editor Michael Tucker or Editorial Manager Anneliese Mahoney.)