
Mark Dangelo: Why Digital Transformation Was Only the Beginning of Continuous Data Auditability Demands
Artificial intelligence solutions are everywhere. As promoted, it is the answer to all changes in business models, it will fix staffing and skill set deficiencies, and it will boost and scale profitability. AI has also ushered in new sets of delivery demands, deployment methods, data operations, and rising expectations in addition to changing roles and responsibilities to define, establish, manage, and retire its rapid-cycle technologies. In short, AI from its cradle to grave is redefining the lifecycles of data ingestion and usage.
For mortgage bankers who have survived the severe 30-year bottoming of the last two years, the hope for a 2025 rising tide to lift volumes and margins is anticipated and rejoiced. Will this be the start of a cycle spanning years of rapid growth? Will the traditional operating principles for origination, servicing, and secondary markets find fertile opportunities in a return to what is familiar? Will emerging technologies like AI have an outsized role in this future—and if so, who will lead, how will it be architected, what will “success” look like?
After 15 years of digital industry transformation based on a quarter-century of industry data standards, a number of BFSI and mortgage leaders anticipate that AI technology inclusion into next-gen business models and operating processes should be viewed as a transition—not another step transformation. The initial challenges of output biases, the scalability moving from prototypes to production, and data reuse linked with cascading data inputs and outputs are believed to be historical and not part of future requirements or design considerations. If markets do recover as anticipated. AI solutions will get credit—but will these patchwork quilt of solutions be an enabler or a false-positive?
Everything in context
AI is at its foundations contains complex, data-driven solution sets that require “data fuel” which is relevant, event-driven, secure, and critically “free from defects.” In simple terms, if the data fuel is dirty, irrelevant, and biased, the AI outputs feeding other AI inputs will create results that may initially excite project sponsors, yet create hidden risks, compliance failures, and legal liabilities. In the end, if the fuel that feeds AI solutions is tainted, it yields misinformation that is cascaded across the enterprise impacting customers and attracting unwanted regulatory attention.
Implicitly, and often hidden within the AI pilots and divergent vendor promises, is the assumption that the data for usage is generally stable, defect free, and readily available. As AI solutions increasingly become interconnected and trained on smaller, internal data sources (e.g., structured, unstructured) the margin of error often rises. Increasingly AI discussions are concentrating on the “intelligence layers” within the AI solution sets, but the risk hidden within starts and ends with the unglamorous data itself.
To put this into focus, let’s look at attributable benefits of audited data used by advancing AI solutions within BFSI and mortgage:
Underwriting: 30% reduction in errors (Forbes) and a 40% improvement in accuracy (PwC)
Margins: up to a 30% reduction in costs (BCG) and across BFSI over $200 million in annual savings (IDC)
Time: up to a 60% reduction in loan processing time (McKinsey) and over 80% reduction in decision making (Deloitte)
Fraud and Risk: AI with audited data can detect over 95% or fraudulent transactions (IBM) and reduce false positives by nearly 30% (Javelin Research)
With loan markets set to rebound in 2025, the leverage of AI fueled by responsibly sourced data will be a differentiator between traditional approaches and the lessons learned from the last three years of pilots and cutting edge solutions. However, while AI algorithms and automation move forward at an exponential pace, the gaps between demands and capabilities are expanding.
Digital standards and transformations were just the beginning
The design of (clean, ethically responsible, and legally compliant) AI data ingestion solutions and elements from the original systems-of-record is not simplistic nor is it a glamorous as designing new AI “intelligence” algorithms. For corporate leaders seeking to embrace AI’s innovative relevance, the idea that additional time, money, and skills need to be allocated to data after 15 years of digital transformations appears counterintuitive.
By discounting emerging data usage across what are familiar data transformation categories—that is structured and unstructured—leaders and their IT operations are not recognizing the vast inputs used by AI solutions. Data auditability beyond structured and unstructured includes:
Semi-structured
Metadata
Dark data
Archived and legacy sources
Event and streaming data
Transactional and reference sources
Third-party, ethically sourced data (beyond data broker inputs)
Derived and AI created (cascading) data
Given the constant change in the data, organizations rapidly adopting AI solutions need to derisk and streamline training and inputs across vast domain-specific sources. Organizations need to objectively assess and continuously audit the data fueling AI solutions, while taking coordinated and comprehensive action to bridge the gaps between current and future data requirements. Where many organizations begin with the features, functionality, and form of their desired AI end-state, the project baselines need to start with system-of-record data origins and existing segmentations contained across department and divisional functional applications.
Mitigating the pains and leveraging the gains
These data compartments represent “pain points” spread across traditional domain systems and encased within legacy infrastructures. As AI progresses, the “data-driven gains” apply new pressures needed by and created from AI inputs and outputs. These pressures at opposite ends of the data spectrum drive emerging, advanced requirements for data audits. An illustration of these elements impacting the purpose, processes, and predictions of a data audit are shown in Figure 1.
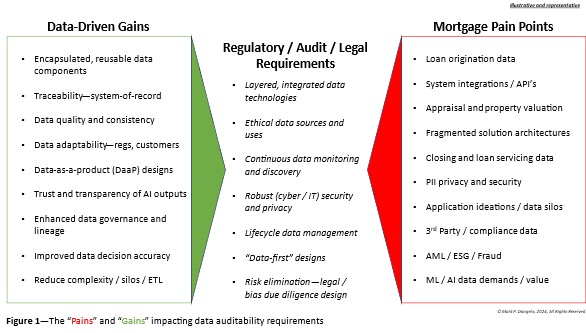
What Figure 1 puts into perspective are the critical drawbacks of non-cohesive AI implementations. If leaders embrace the operating and business model solutions that spearheaded the digital transformation efforts of the last decade, it is probably that fragmentation efforts due to data quality and proliferation will drive up costs (e.g., personnel, power, infrastructure, cloud, et al), inconsistencies, and risks at alarming rates.
Integration of autonomous software layers
To address the emerging regulatory, audit, and legal requirements sandwiched between the “pains” and “gains” of data, enterprises will need to create and deliver against a framework of automated solutions. Each of these building block solutions, built upon a foundation of domain and cross-market data standards, requires tightly coupled stacks of solutions to provide multidimensional interoperability necessary to meet rapidly changing AI technologies that they power.
Figure 2 illustrates the layers of tech matched against characteristics and constraints that will be uniquely defined. Additionally, for each specialized area necessary for continuous data auditability and governance, software leaders are represented to showcase the varied and interconnected solutions that need to be weaved into an automated mesh of audited data requirements.
No longer can data auditability be a one-and-done periodic strategy that provides a point-in-time snapshot—it must be designed for automation and continuous iteration needed for all forms of AI fuel. To understand the complexity and intracity of the data fuel for AI, a deeper dive is required and demanded by regulators, customers, and the very AI technology itself.
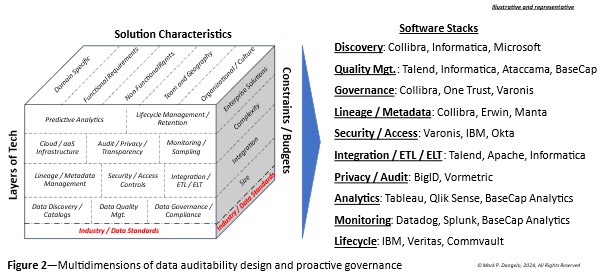
Moreover, Figure 2 represents the granular segmentation necessary to create not just the rigor of data auditability, but also the adaptability of event-driven data and architectures. To compare this against prior efforts of pre-AI data auditability, corporate leaders used a host of specialists and solution containers to define, assess, and implement specialized solutions.
Shifting of roles and responsibilities
These solution outcomes while progressive and managed, led to data auditability “deserts” between one area and another. Moreover, traditional point-developed solutions addressing a unique data component (i.e., lifecycle, analytics, governance) typically are under the control of discrete c-level leaders, each with their own goals, outcomes, and staff. An illustration of these when decomposed further results in Figure 3 with their alignment under the control of distinct c-level executives.
Figure 3 also puts into focus the holistic strategy for software development and implementations that fuel AI—and at its center the need for a CAIO to ensure that software componentization can be stitched together to ensure adaptation.
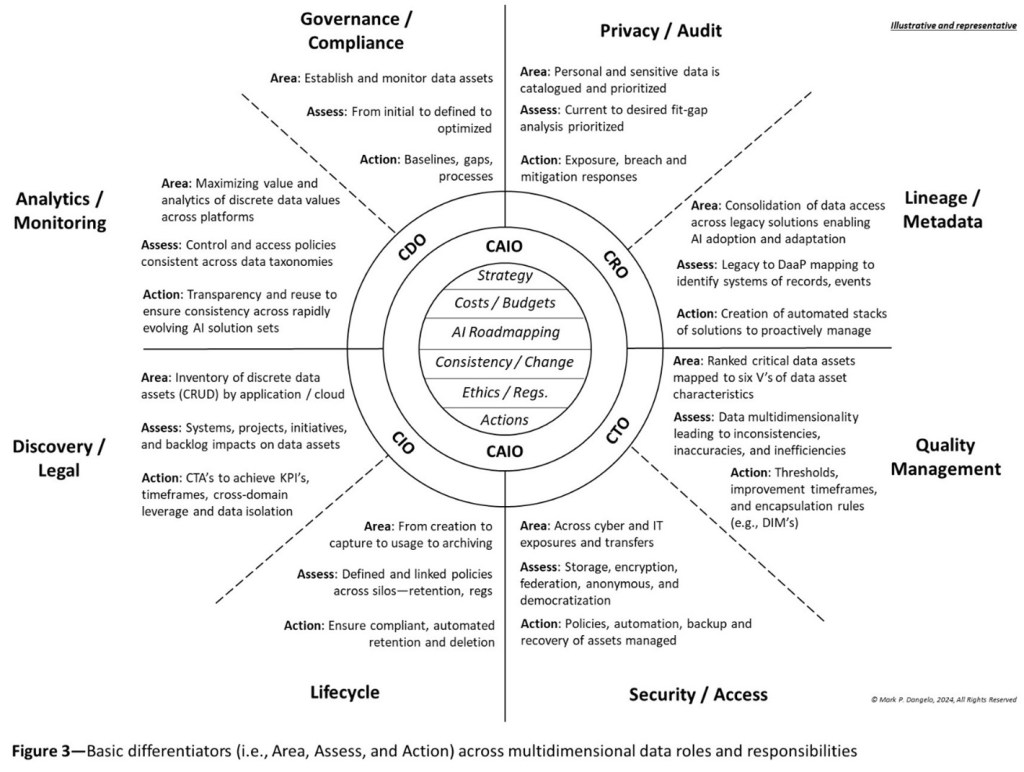
To guarantee that AI is defined, managed, measured, and optimized, the evolving organizational roles and responsibilities must surround shared auditability elements that are shown at the core of Figure 3 including strategy, cost-benefits, change management, and ethical sourcing. By utilizing a holistic approach for data auditability, the negative risks for regulatory compliance, legal exposures, and software actions are minimized allowing tightly coupled solution sets that minimize outside oversight and governance.
The mainstreaming of AI in 2022 promised step shift improvements which in theory eliminate the need and expense for experienced personnel. Yet, as we now comprehend, AI is not flawless, struggles with scalability and transparency, and has yet to robustly address cascading impacts associated with upstream and downstream (data) interconnectivity.
The call-to-actions are contained within the illustrations presented, but in summary they are grouped around:
Cross-domain problem solutions spanning roles, software, and data usage
Atomize the value for each data management solution as part of an active stack of capabilities each interconnected to the others
Focus on reuse and automation to adapt (versus adopt) to vast data sources and uses
Democratize data across a federated cradle to grave data strategy and architecture
Deep data-as-a-product skills and designs ease the burden of data oversight and governance, and
Accept that AI is the next-gen usage for software development that begins with shared data resources, approaches, and rapid-cycling.
In the end when AI fails or succeeds, the data that fuels AI will be the opaque cause-effect lynchpin that starts unintended consequences and delivers value-added capabilities. Without proactive formulation and oversight utilizing the components highlighted, AI will fail to deliver consistently against rising expectations.
With the mortgage industry domains set to rise, so will the risks, complexities, and interoperability demands driven by AI cause-effects. Whereas digital transformation of the last 15 years concentrated around the automation of paper-based processes and data sources, cross-linked AI emerging solutions will demand varied data types, event-exceptions, and recovery capabilities that mark the start of a profoundly different step transformation—not a transition from traditional to intelligent applications.
(Views expressed in this article do not necessarily reflect policies of the Mortgage Bankers Association, nor do they connote an MBA endorsement of a specific company, product or service. MBA NewsLink welcomes submissions from member firms. Inquiries can be sent to Editor Michael Tucker or Editorial Manager Anneliese Mahoney.)