
MBA Newslink Q&A: CloudVirga CEO Maria Moskver Talks AI in the Mortgage Industry
MBA Newslink recently interviewed Maria Moskver, CEO of CloudVirga, Irvine, Calif., about a new whitepaper she worked on regarding Artificial Intelligence in the mortgage industry.
MBA NewsLink: Recently you were one of the authors of a new whitepaper on AI and the mortgage industry. What were some of the key takeaways from this exercise?
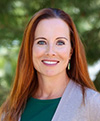
First, let me acknowledge the group of 10 other wonderful authors I worked with to put together the whitepaper, Artificial Intelligence (AI) in Mortgage Banking: Andrew Weiss, Mike McChesney, Todd Luhtanen and Michael Harris from BlackFin Group, Bernard Nossuli from iEmergent, Chuck Iverson from Mason-McDuffie Mortgage, Craig Bechtle from MortgageFlex Systems, Bryan Renton from Citizens Community Federal, John McCrea from MortgageFlex Systems and Jayendran GS from Prudent.ai. This was a team effort and I greatly enjoyed being a part of this group.
After this collaboration, the bottom line is that while there appears to be a substantial amount of buzz and enthusiasm about AI, there is an equal amount of confusion about what it is at this point and how to leverage it both effectively and properly. That fact alone tells me that we are likely several years or more out from wide industry adoption.
AI certainly provides the ability to enhance, possibly even revolutionize, different aspects of the mortgage life cycle through accelerating decisioning, identifying previously unidentifiable opportunities, reducing costs and eliminating manual tasks. The concerns lie with how valuable AI is in our space right now, the likelihood that solutions being billed as AI may not actually be AI, and the reality that the type of data we transact within the mortgage space requires tight governance.
If we don’t know enough about what AI “is,” then how can we be sure our customers’ data and our trade secrets aren’t leaked by these powerful engines to other unrelated applications? We already see regulators contending with the implications of AI on consumer privacy, so implementing AI now likely comes with potential challenges later.
Compliance teams and regulators are skeptical of new technology and new approaches until they have proven to meet regulatory standards and can show that they benefit and not harm the end-consumer. That’s as it should be. Taking care of our consumers is always our top priority. Given the breadth and scope of AI applications, the highest risk with such new technology is its potential for ambiguity and lack of explainability.
Being on both the operational side and now the fintech side of the industry, I experienced first-hand the growing disenchantment with technology both as a business owner and as a provider listening to the market. Many lenders feel that tech vendors have oversold the potential value of their products and that past investments haven’t produced promised results. Lenders need to cut their per unit cost to remain profitable, and AI certainly provides a potential remedy in some areas. But this is also a people-based business, so it is important that we factor in the right places to deploy this type of technology.
Perhaps because my career has spanned both the compliance and technology worlds, within our group, I was one of the more cautious authors. Having seen oversold technology and knowing the potential implications of AI on consumer privacy, I’m cautious about the role AI can and should play.
MBA NewsLink: When you look at AI from your compliance perspective, what do you see as the biggest risks and how do you think regulators will react to them?
The most obvious risk that we called out in the paper is that AI needs to learn by consuming massive amounts of data. In our white paper, we noted that lenders and vendors using AI for decision making will need to continuously curate and audit the data leveraged by AI solutions.
In today’s world, everything we train from, everything we show consumers, everything we leverage for documentation and communication, is governed and regulated. AI allows for the possibility of unregulated information being captured, consumed and relayed. If bad or biased data is encoded into generative AI or machine learning platforms, that could result in harm to both institutions and consumers.
Further, it’s clear that there are limits to testing AI. Not all data is created equal. How are we ensuring that the information delivered and evaluated is authoritative? Yes, we can limit where an engine looks, but these are considerations every lender has to think through as they embark on the AI journey. Simply asking ChatGPT for an answer in an unregulated engine could mean the wrong answer is provided or it is an answer that lacks the regulatory nuance required.
There’s also the possibility that data you enter is captured and leveraged for ancillary purposes we may not be aware of.
Some of the other concerns about AI and compliance raised in the paper include “rogue” LOs using generative AI to produce non-compliant sales pitches and marketing materials and the risks of staffers relying on AI to handle various tasks, rather than following established internal compliance procedures.
There is also the reality that AI in certain applications has shown some level of bias. Considering the amount of work the industry as well as regulators have put into ensuring we limit bias in the mortgage origination process, we must be cautious about believing AI is the final word in both efficiency and fairness. Earlier this Spring, a study by Stanford Law School found chatbots based on different AI platforms might treat black consumers differently based on their names. For example, employment platforms might predict higher starting salaries job candidates with white-sounding names.
This isn’t the first time that this concern has surfaced. Several years ago, one of the large social media platforms settled with the federal government for allowing advertisers to show certain properties to white users and not to users who were perceived to be minorities.
For the past year, the government has been studying bias in home valuation as part of its PAVE initiative. A key finding coming out of this effort is a requirement that lenders take steps to assure that the data going into their AVMs isn’t perpetuating bias.
There’s also a whole section in the whitepaper on risks and guardrails, which includes many of the concerns we’ve discussed here.
MBA NewsLink: Ok, now put on your technology hat: what do you see as the areas where the biggest gains can be made?
Our industry is always looking for new insights that will uncover opportunities to change, and hopefully improve, the way we make underwriting decisions. The rules around underwriting are substantial, but they are also repeatable. What if we could get more predictive? What if we could leverage demographic information and patterns from our collective loan outcomes to know what is the best solution for the customer with the highest likelihood of success?
Using machine learning and deep learning to analyze massive data sets could potentially produce these kinds of “ah-ha!” moments and allow us to establish a more tailored customer experience. We could even start looking at best match-work distribution. There has been a lot of work going on for years on how to match consumers with the most compatible call center agents. Why can’t we think about that in the origination or servicing processes? These kinds of use cases will likely require a level of transparency and auditability to ensure we do not recreate biased processes and outcomes of the past.
Given the sheer number of documents that are needed to complete a mortgage package, using AI and advanced OCR to identify and search documents will most likely be a winning use within the underwriting process, and, in fact, it is already happening to some extent. It’s also a less risky application from a compliance perspective.
Other uses could be to assist with valuations and appraisals, given the amount of property data that already exists. Another potential use case could be for fraud detection; instead of ‘stare and compare’ as we typically do in our industry, AI could more quickly extract and compare data and flag items on disclosures.
Helping our borrowers better understand the origination process, and later the servicing of their loans, is another area in which I believe we’ll see early traction for AI Chatbots.
Despite the educational potential, this possible use case has already come to the attention of regulators who have warned lenders and servicers that they must test for inaccurate information, as well as greater transparency and oversight.
In our paper, we call out potential use cases within every aspect of the mortgage cycle: from prospecting to underwriting to closing to post-close QC and discuss the pros and cons in each area. It makes for interesting reading.
(Views expressed in this article do not necessarily reflect policies of the Mortgage Bankers Association, nor do they connote an MBA endorsement of a specific company, product or service. MBA NewsLink welcomes your submissions. Inquiries can be sent to Editor Michael Tucker or Editorial Manager Anneliese Mahoney.)