
Mark P. Dangelo: AI Progression Represents a Conflagration for Mortgage Technology
(Illustrations courtesy of Mark Dangelo)
AI is everywhere. However, utilizing traditional system approaches to solutions risks repeating the same mistakes and fragmentation from the last 35 years. AI is not a “hammer” or a one-size-fits-all COTS design.
AI’s intelligent data delivery design requires continuous feedback from adaptive learning systems that must collaborate and ingest as part of a cohesive ecosystem of solutions.
Across financial services and mortgage providers, there is one acronym that continues to dominate the headlines—artificial intelligence, or AI. As 2024 is unveiled, AI will expand its beachheads, yet the undercurrents of fear, uncertainty, and doubt (FUD) will grow with 2023 regulatory compliance hangovers that include EU AI Act, White House AI Executive Orders, state AI and data privacy protection restrictions.
However, how will banking, financial services and insurance (BFSI) firms leverage AI beyond the initial excitement? Who will be responsible for their “care and feeding” as they grow and age? And, more importantly, why can’t BFSI retain more than 20% of AI skilled professionals during 24-36 months of employment?
Moreover, mortgage and BFSI leaders while hopeful for AI’s eventual maturity, currently discount it due to increased security, privacy risks, integration and complexity, “hallucinations,” and their nascent commercial introduction (e.g., the AI Goldrush of 2023). While the opportunity for efficiency, speed, cost reductions, and customer satisfaction drive AI forward, the legacy of the past, systemized processes, vendor dominance, and how systems are provisioned are now in flux—or at least they should be.
AI for an increasing number of firms represents the next state of digital transformation that began with the introduction of standards and digitization over a decade prior. The challenge will be how should mortgage and BFSI transition from digital transformation to one that will be dominated by layers of AI-to-AI solutions (e.g., cascading AI, federated AI, generative AI, general AI)?
The priority skills that come—and quickly go
Let’s look at a few of the AI industry statistics that prove discouraging for mortgage and BFSI leaders. The average age of a mortgage and BFSI employee is over 45. Compare that to the average age of an AI expert across the top-10 big-tech providers—under 32. Moreover, the AI mortgage financial services employee 3-year turnover rate is estimated to be between 70% to 80%. The result, the industry depth for highly sought after skills across extensively regulated industry segments diminish an organization’s ability to define, implement, and sustain production capable AI systems.
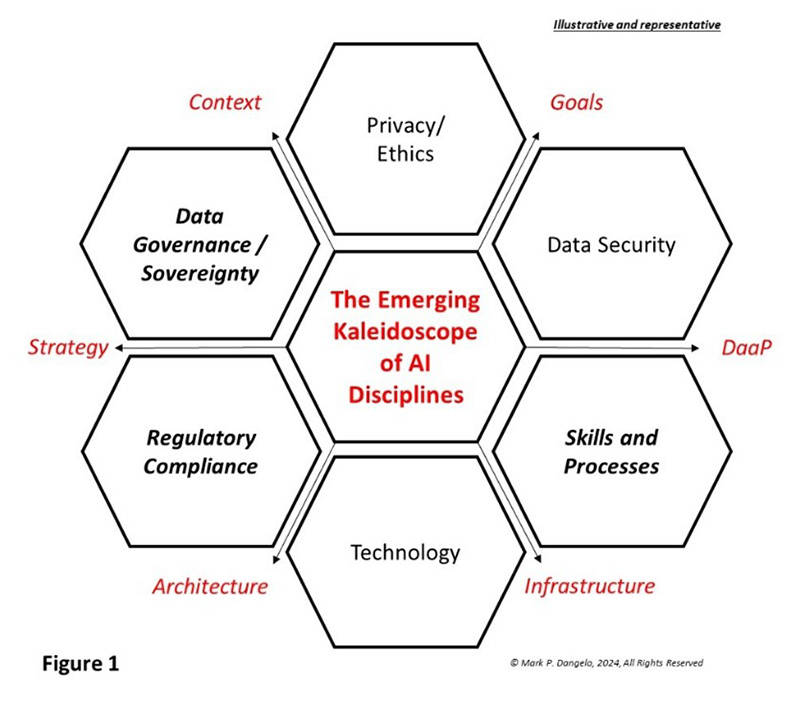
Are the differences of age simply due to expertise required? Are the challenges of turnover due to cultural misalignments or employee expectations? Or, has BFSI yet to determine how to gain strategic and tactical efficacy for data-driven automation (i.e., AI), which is embryonic and lacking in regulatory compliance precedence (and robust use cases)? Now if we wrap these into the mosaic of exploding AI components (see Figure 1), we begin to project why BFSI AI employee turnover might be so high—against a set of emerging technologies that promise to revolutionize every aspect of the industry.
What makes these numbers disconcerting can be found in surveys that show AI awareness has risen just 4% to 8% over the last five years, yet production implementation of AI systems has fallen (versus prototypes and pilots which have by all estimates risen). Are the production declines attributable to regulatory compliance demands, the embryonic and rapidly evolutionary advancements of AI technology, or security demands across fragmented systems each with their own lineage and accuracy?
Today, the traditional systemization of processes has resulted in adaptation challenges for AI solutions, while demanding extensive “humans-in-the-loop” involvement which reduces effectiveness and elongates timeframes.
There is a flipside—what if it is not about AI and its rapidly advancing capabilities, but how industry leaders believe it will implement AI? Meaning, does the mortgage and BFSI industry believe that AI is just another app, cloud solution, or SaaS FinTech that begins—and ends—with system ideations?
Will BFSI and mortgage leaders repeat the same historical mistakes?
There is little debate when it comes to the extensive fragmentation of mortgage and BFSI systems. Their roots of IT complexity sprouted from early computer systems, their application code, and how native paper-based processes were addressed. Traditional systems analysis was about efficiency and speed that paved the way for information engineering, code compartmentalization, and data replication—from warehouses to lakes, from APIs to virtualization, and more recently from cloud computing to data meshes and fabrics. It was the rise of Fintech that provided the greatest benefits since 2008 to BFSI operations, while exposing the fallacy of design thinking that continues to dominate IT system provisioning.
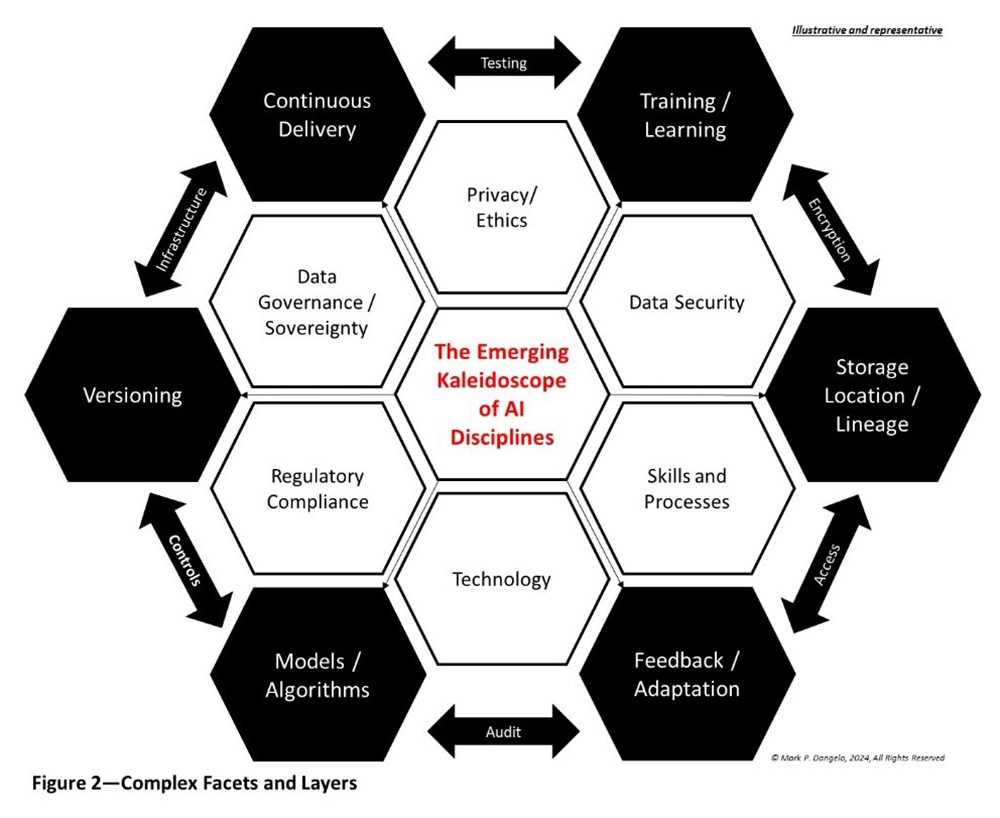
AI solutions are a step function shift from traditional IT provisioning. Whereas the last four decades were about systems, the importance of data grew significantly post Great Recession as organizations struggled with “versions” of data all different across compartmentalized systems. Additionally, the need for volume and throughput took precedence during the last decade. Yet AI, as shown in Figure 2 using the foundational elements of Figure 1, illustrates a materially different approach to creating mortgage and BFSI outputs as a product of data-driven, intelligent, and continually adapting solutions.
The takeaways from the representation in Figure 2 concentrates on: 1) AI is based on a continuously adaptable data design, 2) models and outcomes will never be static as they have to “learn” and be “retrained” to ensure their operational and customer efficacy, 3) there will be many models, designs, and innovations, and mostly importantly, 4) AI will “communicate” with other AI systems creating cascading inputs, outputs, and outcomes that demand continuous monitoring and adjustments. It is takeaway No. 4 that represents a step function shift from tradition.
The question remains, will mortgage and BFSI leaders steeped in tradition of system and Fintech ideation be able to shift their use cases, their operations, their IT investment models, their KPI’s, and even their customer experiences away from what they “know” to what is “possible?” Who will lead, and more importantly how can leaders approach AI across an industry that demands experience, while leveraging AI specialists who average less than half of the work experience found within the BFSI disciplines?
As we map out the kaleidoscope of rapid cycle AI segments against the complex BFSI industry, we begin to interpret the statistics of turnover beyond their initial shock value.
The stage is set—will the mortgage and BFSI industries be able to successfully execute?
As AI revolutionizes many industries and automates complex functions, FUD resonates across workers, leaders, industry groups, and of course, political influencers. While each group has their objections and benefits, the debate is familiar. The failure to shift thinking from traditional to AI data-as-a-product (DaaP) architectures and implementations focuses on causality that may not be innovatively relevant when examining the representative illustration presented in Figure 2.
Now if Figures 1 and 2 are decomposed into more granular components ranked against business value and operational impacts, Figure 3 shows the top 20 AI elements that do not follow the traditional system approaches. Yet, where in the rush to adapt AI are these interconnected components routinely addressed, defined, and actively managed? Figure 3 illustrates the shift from what is familiar to what is required.
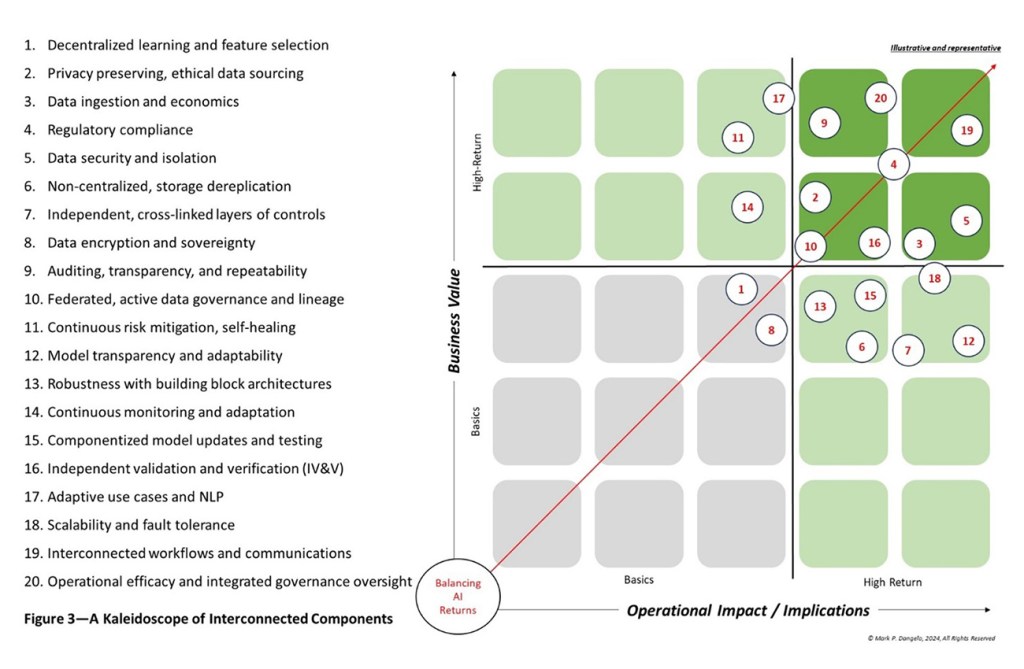
Additionally, this strawmodel representation creates the foundational building blocks that will be consistent as AI technology moves from embryonic deployments to “stacks” of interconnected, specialized solutions that comprehensively alter operational models. If leaders and the disciplines they represent adopt the traditional systems design approaches, every AI system will be process siloed and data fragmented. System implementation history will repeat itself and AI will be a nightmare of fragmented, expensive, opaque data outcomes that cannot be audited.
Now considering the latest AI regulations (e.g., EU, states), the burden of regulatory compliance for the limited AI solutions is viewed as manageable. Fast forward to the end of 2024, and the burden will not be linear as additional, innovative AI initiatives come online. This is why AI deployments require a fresh, tightly coupled set of architectural building blocks before they are selected, which greatly reduces the systemic errors found within today’s traditional and SaaS applications.
Moving forward, AI is rapidly becoming a mosaic of options—a kaleidoscope of building blocks that continually change depending on the innovation and its architecture. A failure to understand the history of creating and provisioning systems represents a larger challenge than skills, technologies, or even stringent oversight—combined.
Those mortgage and BFSI organizations that holistically address the components of Figures 1, 2, and 3 will create new markets and book outsized returns. Those that chase the technology and address the commonalities afterwards will witness rapid diminishing returns for their efforts as they founder from one AI trend to the next.