
Mark Dangelo: AI’s Data-Driven Modernization Requires Industry Reformulation
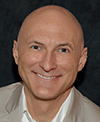
The arrival of 2025 will represent a phase shift for AI solutions. Interconnectedness fueling requirements for risk management, data auditability, and operational efficiencies will expose flaws and limits of AI implementations. For leaders, the journey that is AI will demand prerequisite solutions outside their budgeted initiatives.
After two dismal years, the mortgage industry is looking to celebrate 2025 with rising volumes and improved margins. Underpinned by declines in the federal fund rates and political shifts in Washington D.C., the expectations for a new beginning are shaping products and services.
The current answer to improve performance and meet future consumer needs resides firmly in all forms of artificial intelligence (AI). Recent Bain & Co. research states that nearly 70% of firms anticipate a “high impact” from AI, but currently less than 20% are delivering “concrete value.” Challenges limiting AI’s scale and growth include quantitative impacts, use cases, data, security, and operational integrations.
Yet, the infatuation with all things AI is a journey—not a finite destination. AI requires more than algorithms, unprecedented ethical data quantities, and advanced skill sets. Unlike data transformation in the last 15 years, the movement from application (i.e., process) thinking to data ideations (e.g., DaaS, DaaP, DIM’s) demands architectural and infrastructural building blocks that previously were locked into silos of functionality (i.e., application centric design).
As 2025 unfolds, the realities and requirements of how to layer and connect advancing AI capabilities will take priority. It will be the touchpoints and underlying centers of excellence that will surprise many industry leaders due to the demands for interconnectedness and the error recovery risks and unintentional consequences.
Beyond early adoption—Contextual linkages
Since 2023, AI has become a movement across all operational processes. The promise of faster throughputs, improved efficiencies, and greater accuracy has ushered in autonomous decision making within areas such as underwriting, valuation, customer services (e.g., intelligent AI agents), and credit. Like most innovative advancements, these early point-based application solutions are now being forced to deal with traditional demands of scalability, performance, applicability (i.e., extensibility), and interoperability.
As individual solution excitement gives way to complex operational transformations (e.g., from digitization to data ideation), industry leaders are recognizing that AI is not an end state of independent applications, but a web of interconnected solutions that require foundational enablers to achieve maximum value. The critical themes and solution sets for 2025 will likely include:
Data stack architecture: layers of isolated data access software necessary to manage the explosion of data values—unstructured, structured, metadata, event, et al—linked together but managed discretely (e.g., data governance, cybersecurity, MDM). An example representation would be:
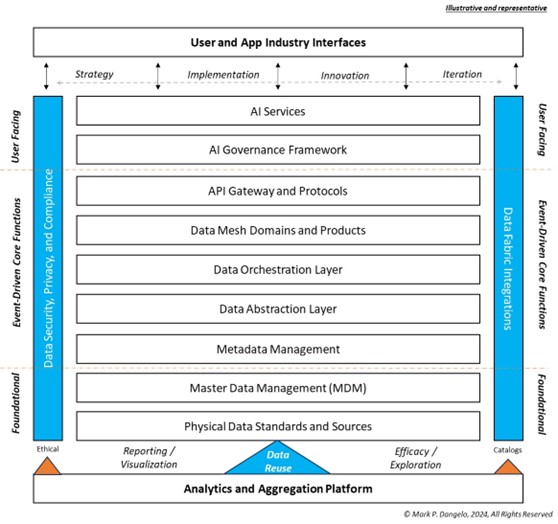
Data transparency and auditability: AI requires not just a one-time training of large-language models (LLM’s) but a continuous adaptation as event-driven data impacts and alters the outputs. When combined with retrieval augmented generation (RAG) to significantly improve industry LLM models, customer and regulator demands for “how” the outputs are derived, manipulated, and shared become paramount to trusting results.
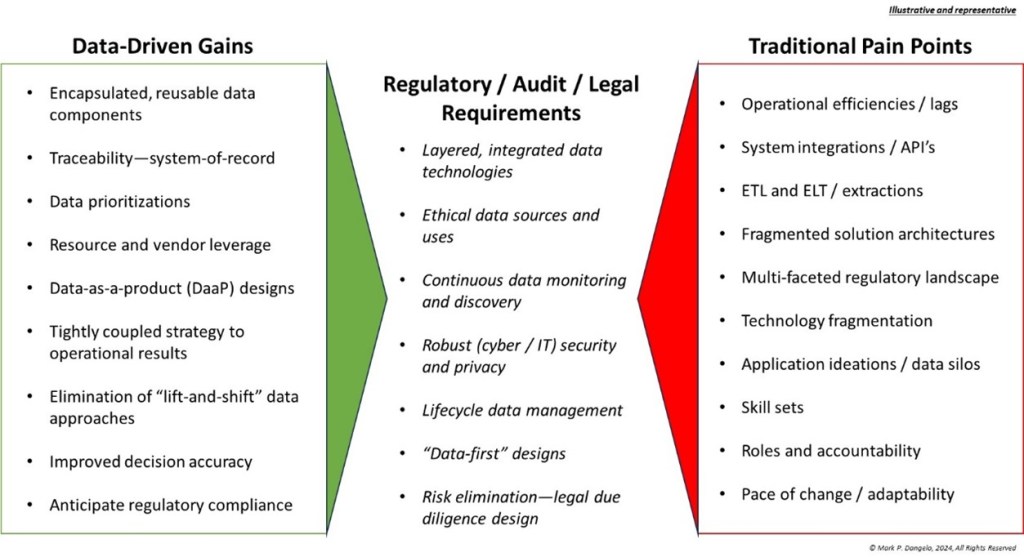
Adaptable use cases: As referenced previously, AI’s novelty and excitement is giving way to a maturation phase that compartmentalizes capabilities into layers of complex functionality that can be mixed and merged to delivery new value to the organization, its consumers, and for proactive regulatory compliance. This requires granular representations that also include use case extensions, acceptance criteria, test cases, and non-functional demands.
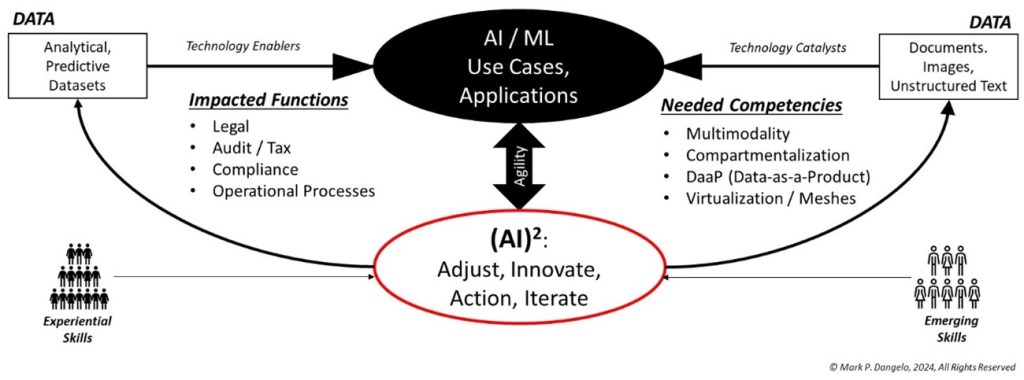
Whereas these themes have been increasingly evident in 2024, as 2025 arrives with different economic and political conditions the quick-fix solution of adopting AI as a remedy is yielding to the reality of what is required. Whereas vendor and internal solution development concentrate on bolting on (generative) AI to existing applications and operational processes (i.e., a digitalization solution approach over robust data transformations), a deeper-dive beyond AI proof-of-concept across the themes necessary for sustainable AI growth tells a different story.
Additive and interconnected priorities
As 2025 delivers against already significant business expectations, the priority agendas needed for cohesive, cascading architecture will increasingly demand multimodal data sources and uses that can be validated—and reused. To migrate away from application centric designs and into data ideation implementations, enterprise leaders who manage the “AI collective” of capabilities (e.g., the chief AI officer, or CAIO) recognize that 2025 is a transitionary year. As part of the organizational transition from application to data ideation the following key success factors will deliver the growth pathways for rapid cycle (i.e., cradle to grave) AI applications.
The demand of standards: AI algorithms are built on data ingestion including data that changes and transforms with events. With more aI systems deployed each touching one another or running in parallel across the enterprise and partners, the demand for consistency, accuracy, and reusability will begin with industry standards including that of MISMO. When linked to off-the-shelf industry applied pretrained LLM’s (e.g., GPT, Watson, Bloomberg) and augmented with RAG (e.g., event data and SLM’s), standards and the data within provide the foundational elements for the above crosslinked design themes.
Explainable AI (XAI): Not to be confused with an Elon Musk AI startup (xAI), explainable AI (large X–XAI) represents the transparency, auditability, and ethical demands that come with robust data governance, varied data ingestion, and output rationalization previously delivered opaquely using COTS solutions. XAI will be linked with Statement of Standards for Attestation Engagements (SSAE 16 / 18) underpinning audit compliance as AI modules come under increased scrutiny from regulators, advocates, and politicians.
Edge computing and digital resilience: With AI cloud solutions growing (as well as their demand for electricity), organizations will distribute workloads to connected devices thereby improving processing times. Moreover, each edge device will be assumed to have “zero-trust” assisting enterprises with explosive risks when it comes to cyber and IT security.
Predictive RegTech (regulatory technology): AI will comprehensively transform regulatory compliance from a check-in-the-box approach to anticipating compliance and future needs. By incorporating the theme designs along with event-driven data, leaders will be able to project with certainty what exposures across local, state, and federal regulations using automated monitoring (with adaptive alternatives).
Collaborative autonomous systems: Whereas enterprises have traditionally had automated system interfaces, the impact of cascading AI systems without a “human in the loop” requires encapsulations, triggers, and monitoring that has limited historical precedents. Critical to these often-federated solutions interacting with one another, is how comprehensive error-recovery will be achieved when systems move a 10x speed faster than humans. How will customers be impacted and what will happen to “undo” AI errors? Conflict and recovery for collaborative AI solutions has been limited to point-based designs.
The explosion of intelligent, data-driven capabilities for the mortgage industry will explode in 2025—that is a near certainty. While industry and research personnel want AI to be simple, that represents an assumption that there is total transparency and recourse even if we use natural language interfaces. AI is not a magic button that operates in a vacuum — industry and researchers have already tried this repeatedly and it is a recipe for future chaos.
One more thing…
With the above themes and the discrete initiatives presented, business leaders must embrace not just the promise of AI, but also the demands what must be continually satisfied and improved to ensure that expectations meet capabilities. However, there is one last item that AI introduces, and which will be unique for every business leader, the models of operation.
Ai as part of a holistic approach to redesigning industry processes and data usage, business model adjustments will be mandatory. To use AI as a fixed solution to improve the efficiency of traditional approaches, would be analogous to putting wooden wagon wheels on a Bugatti. The introduction and expansion of AI interconnected within the enterprise and across partners, will usher in with the rise of volume material shifts in “how business is done” within the industry.
AI will minimally alter revenue streams, change customer engagement models, impact value propositions, alter data ingestion, usage, and retirement, and permanently dismantle the rigid organizational and process segmentations, which have been ingrained across corporate cultures. While failing to integrate disparate technological solutions is déjà vu, the other “50%” of the changes needed by AI reside in the models of operation, organizational structure, and workforce transformation.
The complexity and confusion of AI is just beginning, yet the rush to fast results is bringing back the ghosts of prior step-functional shifts of innovation and computer advancements. The arrival of 2025 seems like it should be all about AI initiatives and advancing technologies. Indeed, it will be. Yet not to recognize and integrate the implications of adoption will deliver returns that are substandard.
In the end, AI in 2025 will be about architectural adaptability — not just products, technology, data, or even regulators. AI is blending of next generation of demands for strategy, architecture, and perfectly aligned to new models of business and human leverage. Those industries and firms that embrace the transformative paradigm will likely come to represent the future of leadership. Those firms that ignore the themes and call-to-action initiatives will face new competitors and a struggle for survival.
(Views expressed in this article do not necessarily reflect policies of the Mortgage Bankers Association, nor do they connote an MBA endorsement of a specific company, product or service. MBA NewsLink welcomes submissions from member firms. Inquiries can be sent to Editor Michael Tucker or Editorial Manager Anneliese Mahoney.)