
Terrell Cassada of LoanLogics: Real Secondary Market Loan Confidence Happens from Within
Terrell Cassada is Executive Vice President of Digital Operations, Architecture and Innovation with LoanLogics, Jacksonville, Fla.
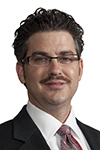
We’ve all heard the saying that real change always happens within. Yet it’s a lesson that has taken the mortgage industry a particularly long time to learn. For decades, the bulk of technological change in our industry has been superficial in nature, and not necessarily within the loan manufacturing cycle, where the real work takes place.
Over the years, there have been countless attempts to make the loan application process easier for consumers and reduce paperwork by adopting online tools and other digital processes—and yet per-loan costs are higher than ever. That’s because true progress was never going to be made by working from the outside in, but from the inside out. It’s only been in recent years that this is finally starting to happen.
New technologies, including AI, machine learning tools and rules-driven workflow, are finally being applied to the loan production process with gusto. Real results are being achieved through automation that drives fewer underwriting touches and exposes quality issues earlier in the production process. And now the secondary market—one of the last remaining components of loan manufacturing still awash in spreadsheets, manual processes, and data inconsistencies—is finally getting its turn at bat.
How Change is Happening
The secondary market is critical for a healthy and functional housing industry, as the ability to sell loans to investors frees up capital that is used as fuel to make more loans. However, the process of selling and onboarding loans can be tedious, and there are many inconsistencies in how institutions treat loan files and their underlying data. For practically its entire existence, the secondary market has been plagued by these inconsistencies and an overall lack of full transparency for loan file data quality that have made the processes of loan trading and loan onboarding a cumbersome task.
Fortunately, the advent and implementation of artificial intelligence (AI) and machine learning tools have proven capable of transforming this scenario. When combined with optical character recognition (OCR) data extraction technology, AI and machine learning can significantly speed up the loan trading process by automating countless manual tasks. In addition to lowering costs, these technologies can also help servicers and investors gain greater insights into their portfolios for trading decisions, especially when determining where risk lies and which loans to sell versus which loans to retain.
Perhaps the biggest benefit of these technologies is that they reduce the overuse of spreadsheets in the secondary market. Spreadsheets are notoriously time-consuming to use and prone to errors due to manual data entry, inconsistent formatting, and limited functionality. Even though there is more loan data available than ever, mortgage companies that are slow to adopt data-driven automation have limited its use by trapping it in spreadsheets with little data validation to ensure its accuracy.
By contrast, machine learning, data extraction algorithms and rules-driven technology can analyze loan file data more accurately and consistently, reducing the risk of human error. This eliminates the historic “stare and compare” methods of checking data and loan quality, which greatly reduces the time and overall costs of due diligence. By greenlighting the vast majority of files in which the data can be trusted, companies can focus on exception-based processing, which allows them to move loans forward much more efficiently.
Improve Confidence and Pricing
Another benefit of these technologies is they introduce standardization that accommodates variations in how different investors look for different data elements when determining risk. Machine learning can be trained on critical and non-critical document types, fueling better data extraction that addresses the broadest set of investor requirements. Subsets of documents and data elements can then enable consistent delivery to an inconsistent landscape of investor requirements.
If an investor needs to check 30 critical document types with corresponding critical data elements when buying a pool of loans, they can rely on machine learning to classify documents correctly so that data extraction programs and rules-driven data validation tools can then do the heavy lifting. Rather than spending several days poring over loan files by hand, investors can narrow their focus to exception management, significantly improving loan file review turn time.
Machine learning technologies do this by leveraging confidence scores, which reflect the likelihood that the results returned are correct. For example, if it’s critical to get certain documents in a loan file correctly classified, a large sample of accurate documents for training can drive up confidence scores. A threshold could then be set to only “pass” documents with very high confidence to ensure critical documents are always prioritized for accuracy. Other non-critical documents might have lower confidence score thresholds to allow more to pass through automation without review.
Increased confidence that loans in a trade meet investor requirements also translates into higher margins. Fewer exceptions found may result in better pricing for the seller, while reducing the time and effort it takes a buyer to assess loan pools for purchase reduces operational costs and increases competitiveness by optimizing time to fund. Furthermore, automation has also facilitated the emergence of new loan trading strategies and marketplaces that were previously unavailable to investors.
Many secondary market professionals might not realize that a variety of machine learning enabled exchange platforms are being used today by entities ranging from MSR brokers and capital market firms, to the GSEs. Reliant on technology to do the heavy lifting, these networks standardize seller loan files to match the stacking order and data requirements of many investors. As a result, investors can access a wider range of loan opportunities and diversify their portfolios, which in turn can lead to higher returns.
Smoother Onboarding
One of the largest issues whole loan trading and MSR markets face is the cumbersome nature of the actual transfer and the onboarding process to servicing systems. The onboarding process is time sensitive, and if mishandled, it can not only have an impact on the trade but create downstream dissatisfaction from borrowers.
Fortunately, AI and machine learning can also help to streamline the document management process during loan boarding. Servicing system taxonomy can vary, which brings us right back to the value of standardization. To prevent delays, loans coming from a variety of sources can be funneled through a process that creates predictable naming, classification and data fields. In other words, mapping only needs to be set up once to facilitate smooth onboarding.
Once this happens, relevant critical loan documents and data can be quickly and accurately identified, shortening the time it takes to onboard new loans and making it easier for servicers to scale with market opportunities. This can also reduce or mitigate the risk of missing or misnamed documents, while improving compliance for timely onboarding and borrower communications.
Proven Results
Look at the largest correspondent lenders in the industry and you will find the impact AI, machine learning and automation are having on the secondary market. Years ago, these tech-savvy institutions began looking for new technologies to classify documents and extract data for their automation initiatives. At that time, correspondent divisions were experiencing a wide range of file delivery issues involving missing documents, incorrect document versions, documents that weren’t signed and mismatched or inaccurate data.
Ever since then, machine learning has been coming to the rescue for correspondent lenders coping with loan documentation discrepancies and increasing needs to enhance data automation. The maturing of these technologies and the training that has gone into improving accuracy through confidence score analysis is enabling the recognition of an even larger number of accurately classified document types for greater levels of data extraction.
These leading lenders continue to drive growth using substantially more automated rules that reduce manual tasks and focus on rapid exception clearing, which reduces adverse pricing charges while eliminating funding delays and holdbacks. New use cases powered by loan file data are also making significant strides in helping to power downstream systems and continually improve countless internal processes.
Commitment to Change
It’s true that we’re finding real change from within beginning to take place. But the reality is that some lenders are ahead of the game, while others are further behind in technology adoption. Many addressed the high volumes we experienced in recent years by overstaffing rather than automation. Given current volumes, the impact on the cost to originate has been stunning—and not surprisingly, we are seeing right-sizing taking place across the industry.
The past year has been a painful process. But the silver lining is that the technologies put in place today will enable organizations to scale as needed when the market returns to normal. Overstaffing no longer needs to dominate every aspect of the work we do.
By leveraging the power of AI and machine learning, sellers can be confident in the accuracy and completeness of loan files so they can take advantage of market timing to maximize loan value. Investors can now achieve greater insights into the loans they are buying, improve their ability to scale, and make more informed trading decisions with improved data accuracy.
As we look to the future, change from within across all sellers and buyers in the mortgage industry will enable a more efficient and healthy secondary market with downstream benefits for borrowers. That’s the sort change I think everyone can get on board with.
(Views expressed in this article do not necessarily reflect policies of the Mortgage Bankers Association, nor do they connote an MBA endorsement of a specific company, product or service. MBA NewsLink welcomes your submissions. Inquiries can be sent to Michael Tucker, Editor, at mtucker@mba.org.)