
Sponsored Content from InRule: Transform Mortgage Lending and Real Estate with Machine Learning
Machine learning (ML) is playing a significant role in the transformation of real estate and residential mortgage lending. It increases productivity by automating decisions. Machine learning also reduces risk and error rates, while increasing the decision consistency — versus relying on human interpretation and discernment.
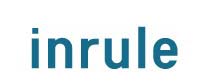
However, it can be tricky to get ML right. It’s important to consider an approach that allows you to manage and organize your decisions in a cohesive manner and provide guardrails so you can avoid the potential unintended consequences of an algorithm gone awry. That’s when it becomes valuable to pair machine learning with a decision platform for decision and business rule automation.
But even before you dig into tools specific tools and technologies, it’s essential to first ask the right questions to create the right scenarios for ML.This can be challenging; it requires a deep understanding of nuances. For instance, a single prediction might impact several scenarios.
Nevertheless, walking through a known set of scenarios is a great way to jump-start creative thinking, laying the groundwork for a project.
At InRule, we spend a lot of time discovering and describing scenarios with our customers. The challenge is to reflect on the relevance of these scenarios and make sense of them through the lens of specific operations. With that in mind, we hope this article helps you examine and consider scenarios for your enterprise — and to identify ML project opportunities.
There are many factors driving adoption of ML from a decision automation point of view. Some of these factors are externally driven by markets and competition, while others are technology oriented. Out of the many factors others have written about, at InRule we believe the five presented below are the most salient.
What’s Driving the ML + Automated Decisioning Push?
Self-service. Homebuyers want to do more on their own and expect the user experience to deliver on their goals. If they provide their information, they expect a return in immediacy, completion, and transparency. Combining ML and decisioning reduces cycle time and paves the way for straight-through processing.
Accessibility. ML is more accessible than in the past. A trove of new AutoML offerings are entering the market, driving down the cost and time-to-value of operationalizing models in production systems. AutoML allows business analysts to train ML models safely, without the need for a data science degree.
Operationalization. An ML model on its own is not very useful. It must be deployed as a service or used within an application (or a batch process) to make an impact. Decision platforms deploy as services and easily incorporate ML models into the lifecycle, giving them governance and a well-known set of team practices to draw from.
Data. Real estate companies and lenders have more data than ever before and are ready to use it. To get the most from their data, they often rely on a Chief Data Officer (CDO) to drive data strategy and operations. We believe the CDO role naturally aligns with decision management as an operational focus.
Explainability. ML is more capable of explaining mathematical relationships between data points and how they behave in a prediction across segments. ML, combined with decision automation, makes it possible to simulate and influence KPIs like never before.
Scenario #1: Residential Sale Price Prediction (Algorithmic Appraisal or AVM)
There are several uses for a reasonably good residential sale price prediction. While Automated Valuation Models (AVM) have been around for a while, they have limits unless they have been modernized with ML and trained with more features. We have spent a significant amount of time on AVM prediction and continue to be surprised by the number of scenarios it can improve by circumventing problems long before becoming a downstream issue in the lifecycle of a mortgage loan. As the data footprint expands among lenders and ML techniques become more accessible, we expect to see AVM move in-house over time. Lowering the cost of AVM opens the door to expanded use. Let’s break down several areas it can impact:
- The conflict between seller and listing agent. During the process of listing a property for sale, both the seller and the listing agent want to understand the relative value of the property. The seller wants to know what the price should be and wants to avoid conflict with the listing agent pushing for a lower price. Review of recent comparable sales remains a standard practice for pricing, but a good ML model can come within a low margin of error; for example, Zillow states the Zestimate® is within a 4.3% median error rate.
- What-if tools. The listing agent knows a relatively high listing price will affect how long the property may be on the market. A good model forecasts the sale price relative to the number of days on the market and the property’s characteristics. With ML used as a forecasting tool, the listing agent can easily demonstrate how price affects days on the market.
- Borrower comfort. The borrower’s prequalification for a mortgage loan is dependent on the property’s appraised value, Loan-to-Value (LTV) ratios, and cash required to close. Calculators using price prediction improve the buyer’s experience by educating the borrower before committing to the next steps in the journey.
- Early detection. A lender’s underwriter may hold up a loan application if the appraisal is inaccurate. AVMs using ML and rules to value property characteristics can detect valuation problems and route the application accordingly before it reaches underwriting.
- Vendor management. The appraisal is the longest running task in the origination process. Inconsistent and unsupported valuation from appraisal service providers slows the underwriting process as new information is requested. AVM, by measuring the difference between a predicted value and the manual appraisal, can identify problems early and over time determine the quality of service providers.
- Portfolio fit. Investors would like to predict property valuations to judge the risk in their portfolio and pre-select loans in flight that fit specific parameters. Investors might also look for real estate value predictions to focus on specific micro markets for investment.
- What to build. Builders want to understand which features net the most value for a given market. With the addition of ML and decisioning, they could discover that some features simply add cost, negatively impact profitability, or add time on the market for a new home and use this information to build a more attractive portfolio.
Scenario #2: Portfolio Retention
Loans within a portfolio are affected continuously by borrower circumstances and market conditions. Predicting when a borrower is likely to refinance allows the servicer to make offers and hopefully keep the loan in a portfolio — even if it is under new terms. Conditions such as low interest rates, rapid growth in value (perhaps detected through algorithmic appraisal affecting LTV), remaining loan period, and employment statistics can influence refinance candidates. Forbearance/foreclosure candidates are also within scope for ML models.
Scenario #3: Automated Underwriting System (AUS) for Capital Markets
Loans sold into capital markets need an AUS to augment underwriting to the specified program guidelines. Decision automation delivers the bulk of underwriting automation technology (logic represented as rules). Combining a rules-based approach with ML provides a complete AUS capability that can grow over time and specialize when required. If you have built your AUS in-house, there is sufficient room to differentiate and improve operations beyond the capabilities of a third-party system. Consider implementing conforming criteria as a baseline while building out improved predictive models covering problems up front and tailor fit for a specific portfolio.
Scenario #4: Loan Program Selection
Currently, most digital transformation initiatives focus on self-service experiences. The goal is to improve the customer experience and, at the same time, decrease the loan origination cycle. Most Loan Origination Systems (LOS) were designed around the loan officer and back-office staff, not the borrower. Because of this, LOSes typically have multiple qualifying loan programs for a given application. It’s the job of the loan originator to offer relevant products based upon the needs of the borrower. Borrowers are not all the same, and their application can trigger unique underwriting requirements. Often the borrower’s goals contradict or balance against each other as constraints. Borrowers want the best loan terms that fit them. And fit is more than the estimated payment and transaction costs. Other factors, like time to close, lender responsiveness, and access to self-service tools, improve fit.
Borrowers want to feel like their selected loan option is the right choice. The challenge for self-service is to dynamically walk the borrower through scenarios and quickly eliminate products that don’t work. An automated, dynamic questionnaire, driven by rules, can narrow scenario factors before an ML model offers loan programs tailored to a specific fit.
However, to optimize resources and maximize the self-service experience, it’s best to apply additional algorithmic strategies until only a small percentage of borrowers must interact with someone in real time. Borrowers abandon experiences that do this poorly. A lender’s ability to succeed at self-service is disruptive to the industry, as demonstrated by the mobile experiences from top nonbank lenders in the United States as they continue to capture market share.
Scenario #5: Propensity to Close (Loan Abandonment)
While borrowers abandon loan applications for many reasons, let’s examine a common scenario: A borrower locks in a rate during a volatile period. While they are waiting for loan approval, interest rates fluctuate by a half percentage point or more. Rather than renegotiating the terms of their locked rate with their current lender, they select another lender with an offer at a lower rate. The first lender only discovers the loan abandonment when calls made to the borrower are not returned and it’s too late to recover the application. Early detection of, and responsiveness to, abandonment potential drives closed loan volumes above the normative pipeline activity for the industry. Areas of focus could be:
- Automatic monitoring of a borrower’s credit report for additional pulls after a rate lock period has begun
- Tracking borrower engagement with portals and communications
- Building ML models that correlate borrower population behavior against rate fluctuations to define rate thresholds for automated alerting or pre-emptive action
- Detection of behavior in early-stage leads makes the funnel more predictable by ranking the amount and type of data collected. Modern portals using ML with automated decisioning not only fast-track borrowers but can deliver multi-channel responses to save at risk loan applications.
Next Steps
Spend time with your team of stakeholders on these scenarios. Also try to imagine other scenarios not mentioned.
And if you are analyzing a business process, think about what you would do differently if you knew something was likely to happen. A model on its own is not useful unless it can influence business outcomes. Some risk in a loan origination process could be mitigated with a predicted value of a property. Combining the prediction with action is the challenge. In short, look for predictions that flow easily into a series of actions the team understands.
Examine existing KPIs within your operations. If you already measure some aspect of the process, it’s a lot easier to understand results within that framework. You don’t have to overthink the objective. You can simply try to improve what you are already doing.
Be prepared to augment your data from multiple sources. Increasing the data footprint increases signal for predictions. Once you understand the mathematical relationships in the data, remove the superfluous aspects. If your problem can be segmented to a smaller set, that’s even better. Work with your team to inventory data as it is, shoring up the gaps.
Consider who on the team is in the best position to work with data. Rule authors work with data all the time (not just the transactional data). Which of them has a degree that uses data science such as economics, psychology, or sociology? The foundation from their academic background might be enough for data cleansing and experimentation with AutoML (rather than writing Python to develop an ML model with code). If an outside consultant is needed, validate their track record of getting ML models prepared for production use. Rely on your consultant if you have trouble selecting an ML approach to your business problem.
Finally, it’s important to note that many initial ML projects fail because the team developing the models lack sufficient operational practice to impact the organization. They know their discipline, but are missing methodology and technology to safely socialize, deploy, and govern the model. Moreover, ML is excellent at prediction and detection, but cannot take action on its own. It requires a foundational technology like decision automation to take action within a production system — also referred to as operationalization.
In fact, decision automation by its nature operationalizes both decision models and ML modes by providing a complete set of team features, and governance while deploying as a service. You get more out of both technologies when they are implemented together.
Transform Your Decisions, Transform Your Business
Empowering teams with the ability to automate decisions helps ensure the business is making the right investments for the future. Writing and managing those decisions — without the need for complex code modifications — while embracing ML can transform both the business process and the customer experience.
Want to see just how far automating decisions can propel your business? Schedule a demo with InRule today.
InRule’s customers include many of the largest lenders in the world. In fact, more than half of the loans processed by the top eight originators in the U.S. and more than one-third of the mortgages processed by the top five lenders in the U.K. are powered by the InRule Decision Platform. We offer end-to-end guidance and technology to solve lending problems. We’d love to connect with you. Contact us at info@inrule.com to learn more.
(Sponsored content includes material submitted independently of the Mortgage Bankers Association and MBA NewsLink and does not connote an MBA endorsement of a specific company, product or service. For more information about sponsored content opportunities, contact Bill Farmakis at bill@jlfarmakis.com or 203/834-8832.)