
Gen-AI and Mortgage Lending–All Hype or All Real? Francesco Paola From Mozaiq.ai
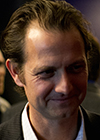
Francesco Paola is a business executive and technology entrepreneur with more than 25 years of global leadership experience in enabling enterprises to adopt innovative and transformational technology solutions at scale. He has spent his career delivering technology strategy and implementation services at the forefront of innovation, including deploying some of the first ecommerce solutions in financial services, developing innovative payment platforms, enabling adoption of outsourced technology and business services, helping enterprises adopt the transformational power of cloud computing and most recently enabling proliferation of intelligent automation solutions in the mortgage lending ecosystem.
Gen-AI and Mortgage Lending… given the daily carnage of Gen-AI failures, one has to wonder.
A META Gen-AI chatbot (now decommissioned), trained on scientific research papers, made up purported academic papers and generated content about the. . . history of bears. . . in space.
A World Health Organization (WHO) Gen-AI chatbot that made up names and addresses of non-existent clinics in the Bay Area.
Google’s Gen-AI engine recommended the use of “non-toxic glue” to solve the problem of cheese not sticking to pizza. The answer for this query appears to be based on a comment from a decade-old joke thread on Reddit. But the LLM was trained using this thread as source data (more on this later), so the answer… stuck.
And McDonald’s just shut down its partnership with IBM, whose watsonx Gen-AI technology powered McDonald’s drive-through automated order taker. How about some bacon with that ice cream? And your order of 260 chicken McNuggets is coming right up.
That’s the fundamental problem with chatbots: they will always hallucinate, sometimes correctly, sometimes incorrectly—they’re engineered to make stuff up. Why?
A Statistical Inference Engine
By now we all know that the underlying engine of a chatbot is called a Large Language Model (LLM). The challenge is that an LLM is not a database, nor a search engine. It is instead made up of a bazillion (lots of billions) numbers that are crunched to calculate responses.
The numbers in the model (think of it as a gigantic spreadsheet) are set when the model is trained, and an LLM requires infinite amounts of data to be somewhat relevant, and somewhat accurate.
A chatbot creates word sequences from scratch every time a question is posed, because these bots create the output text by predicting the next word in a sequence: a Gen-AI powered chatbot is a statistical inference engine. It asks itself: what is the statistical likelihood that the next word in the sequence “I walked in the ___” is “park” or “room” or “door”. Or “tar pit”? The word with the best statistical score wins. And the score is determined by the quality of the data used to train the LLM. Biased data delivers biased answers. Toxic data propagates toxic answers. Which is why smart companies implementing Gen-AI chatbots require that an answer be traced back to original source data, and why results must be critically analyzed by humans.
So, an AI chatbot based on an underlying LLM hallucinates all the time in order to concatenate words in a logical sequence. Many times it’s right. Sometimes it’s wrong.
Increasing the Accuracy
LLMs can become more accurate as they are continually trained with more data, and are constrained in scope. How does that work? A friend of mine at a major financial services institution is training an LLM with targeted, proprietary data. This is called Retrieval-Augmented Generation, or RAG. Specifically, the firm used a set of internal documents (“external data” in the context of the LLM) to train the LLM, with (human) subject matter experts querying the LLM and providing feedback with prompts as simple as a thumbs up or thumbs down, by typing in the correct answer (if the chatbot spat out garbage), and by going into the internal documents and modifying and optimizing the text (the source data) i.e., making the answer less open to interpretation and more exact. This is one way to create a chatbot that is “smart” enough in order to achieve a high confidence level in their answers. The external data augments the underlying LLM by being converted and stored in a vector database—even more numbers! Then, based on the query, the chatbot will return the most relevant answer, using this external data as the main source of information to formulate the answer.
OK, fine, so now the chatbot is more accurate. But regardless, people will get careless as the accuracy of LLMs improves, and when errors do happen, users are more likely to miss them. And errors will continue to happen.
Gen-AI and Mortgage Lending
The mortgage lending fulfillment process cannot afford errors. Consumers will be negatively impacted by the inherent bias. Lenders’ already thin margins will shrink, and their reputations will be sullied. What then are safe uses of Gen-AI in mortgage lending?
Summarization and Synthesis: for borrower loan files, accounting pronouncements, appraisals, financial reports, financial news impacting policies, processes, and reporting. For example, a common use case is in the call center: other than the obvious call routing and agent prompting (nothing revolutionary here, it’s done today with legacy technologies), call summaries can be auto-generated by the Gen-AI engine (trimming up to 40 seconds per call), with increased accuracy, and the ability to trigger follow up actions.
Deep Retrieval: augment IDP solutions with Gen-AI to extract information from unstructured data sources. For example, Google (the same one with the cheese and the glue) has deployed a Document AI solution that has Gen-AI embedded into its platform. The platform enables data extraction from scanned documents and does not require hundreds of documents in order to be trained. With unstructured documents that have multiple variations—like bank statements—with mostly simple key value pairs, the Gen-AI-powered data extraction function requires 95% less training and quality control than what a Machine Learning-based (ML) model would need.
Loan Officer and Broker Support: a specialized (RAG-ged) customer service chatbot (notice I didn’t say “underwriter support”; see hallucination notes above). A targeted chatbot allows loan officers and brokers to summarize the salient borrower information (minimizing the task of pre-compiling paperwork) and send the summary to the lender account executive to determine whether a borrower even falls within the qualifying parameters of a loan product.
If trained further, the chatbot can also offer suggestions on how to structure a loan that falls within valid qualifying parameters if the borrower does not qualify for a loan product that was initially offered e.g., the chatbot analyzes the borrower’s loan file and sees that she has an account (type or balance) that could counter the deficiency present in another area.
And, one can apply the RAG concept to train these Gen-AI models; lenders have the data, from historical and current loans, in their systems of record, and from document indexing and data extraction from loan documents (assuming these lenders already have foundational automation deployed).
Conclusions The moral of the story: there are use cases in mortgage fulfillment and servicing where Gen-AI delivers tangible benefits without compromising security, accuracy, and the lender’s reputation; one can trust, but one must verify. All the time. And if Gen-AI is deployed, then ensure that it’s done to augment the tasks of the lender’s human resources, and never the tasks of consumers or homeowners, especially offering mortgage advice or loan decisioning, with the goal of making the human resources more efficient. And that the human has the last word.
(Views expressed in this article do not necessarily reflect policies of the Mortgage Bankers Association, nor do they connote an MBA endorsement of a specific company, product or service. MBA NewsLink welcomes your submissions. Inquiries can be sent to Editor Michael Tucker or Editorial Manager Anneliese Mahoney.)