
Mark Dangelo: AI and Aging Workforces–Not Mutually Exclusive
The spotlight on artificial intelligence (AI) is both an Angel and a Demon. The changing value for industry pathfinders and system architects resides not only in securing efficiencies and insights, but in its robust amalgamation with deep domain knowledge workers who are skilled in cascading complexities. As our workforces age, it is the opportunities at the other end of the spectrum which will deliver sustainable differentiation for BFSI and mortgage operations.
In real estate the mantra is about location, location, location. Today, it is about AI, AI, AI. There have been hundreds of billions invested in the last 24 months with billions announced in the last 3 months in chip development, in software, in algorithms, and of course, in data. Yet, the focus of scaling emerging AI capabilities in bank, financial services and insurance (BFSI) and mortgage should be beyond algorithmic and into the deep domain verticals leveraging experienced personnel and their nuanced skills.
Artificial intelligence (AI) has many discrete meanings, implementations, and even configurations. It can be cascading (i.e., AI talking to AI), interactive (i.e., chatbots), or even automating digital and paper-based processes (e.g., RPA).
Yet, the many faces of AI are not singularly about younger, generational workforces and their embrace of rapidly exploding machine intelligence, but how AI can also leverage experiential domain knowledge encased across years of learned execution. The use case(s) of leveraging experiential knowledge holds the greatest profit potential as workforces continue to mature, retirement age rises, demographics and immigration shift, and costs of hiring freshly minted AI engineers explodes (WSJ, >$1MM packages).
To frame the discussion of AI impacts and value propositions, we need to start with the average age of the U.S. worker. It is now over 41 and increasing, while measured AI functionality is experiencing exponential advancements. Additionally, per published trends the average age of a BFSI / mortgage banker is 40+ for nearly 70% of the industry personnel.
Now factor in the finance and mortgage industry have experienced material challenges from rising interest rates and political divisions all fueling future risks and uncertainty. Moreover, employee apprehension of how enterprises intend to leverage AI in their daily work tasks (per Oliver Wyman) indicates that nearly 70% feel tense and nearly all feel that their efforts (in the face of AI) really don’t matter to their leaders.
These foundational realities, coupled with AI expectations, are fueling business strategies, architectures, and software solutions that indicate a bifurcation of workforces favoring younger workers. That assumptive axiom—age as the causality for AI advancement—may prove to be fatal.
Today’s and Tomorrow’s AI is so “Last Season”
AI advancements today and projected forward have the potential not to only add trillions of topline (ranges vary from McKinsey near $4 trillion and Oliver Wyman near $20 trillion global GDP), but also to supercharge workflows, supply chains, and usher in the next series of data transformational and digital advancements. It appears in the last 24 months of AI progressions, that AI is the next-gen “glue” that was missing from shifts of business and operating models, for future product development, and rapid-cycle innovations.
AI has captured the consumer’s imagination, corporate budgets, and more importantly competitive differentiators promising superior margins. Yet, business and HR leaders must adhere to other tangential demands and regulations against AI bias for age discrimination, ethnicity, social status and even incorrect decisioning due to digital content.
Stated clearly and painfully, for many seeking opportunities in a post-Covid workforce rebalancing, AI and ageism in the workplace is like saying the quiet part out loud. And, whereas the current model of piloting and scaling AI focuses on the new, its comprehensive and scalable opportunities reside with iterative deployment across domain workforces.
AI in the hands of professionally trained and reskilled domain experts will not only improve margins, but also mitigate risks, expand data quality and usage, and provide analysis and innovation that is more than just a “destination” solution. Yet, where should forward-looking teams be looking beyond the hype? Where are the opportunities to use AI with experienced workforces?
Rather than use experience or even unproven academic research, why not ask AI where AI in the mortgage and financial industry is best applied? Asking the common AI chatbots, the list they offered included the following segregation of what is today—and what still is evolving.
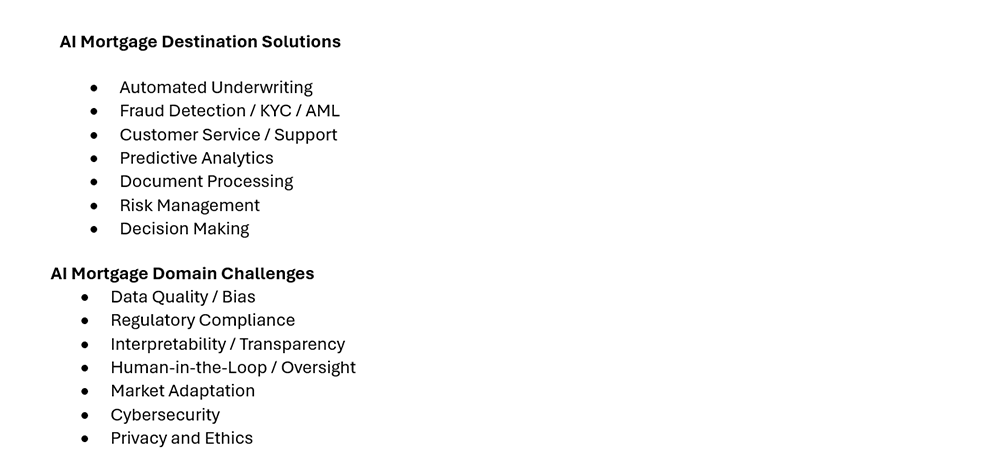
Using the aforementioned, are these the starting points for effectively deploying evolving AI solutions within industry verticals that require deep domain expertise across highly regulated products and services?
AI Makes Experience More Valuable
For vendors and outsourcers, the answer for AI resides in their product roadmap offerings. For consultants and transformation specialists, the answers reside in processes and organizational alignments. For BFSI leaders seeking sustainable change, the answers reside in achieving a set of baselines as foundations to design, implement, and maintain AI as it undergoes hypercycle changes across multiple domains and architectural designs.
Where to begin? Let’s use a common system analysis methods of “radar” charting to assess capabilities and demands across multiple aspects.
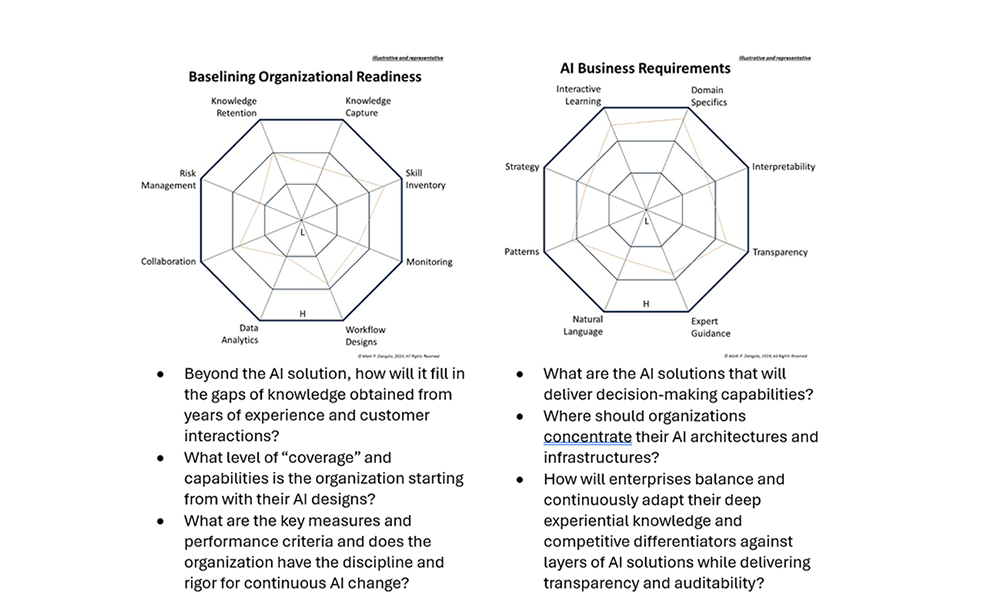
The above radar charts above provide a rudimentary starting point to match AI advancements to compliment, leverage, and grow utilizing deep industry knowledge. However, the next step of the journey to match AI to the workforces requires a specific review and projection for domain verticals already accepted and what constitutes “AI at the edge?”
From Baselines to AI at the Edge
Across BFSI and mortgage operations, the early pilots and future designs focused on process efficiencies. The focus was on digital data—centralized, distributed, decentralized—and the transformational impacts when algorithmic models were applied. The designs were driven by needs of efficiency, time-to-market and replacing entry-level skills.
These models were then expanded pushing into more traditional capabilities that are shown on the left-side radar chart below delivering not just automation, but also insights. These insights continue to be expanded with rapidly iterating machine intelligence and large language modules, yet the intersections of designs and datasets have unleashed advanced potentials that extend between AI point-based capabilities. This rise and unbounded future potential are where emerging demands and requirements are pushing the requirements to not replace older workforces but to reskill, retrain and grow the deep domain, multimodal human intelligence.
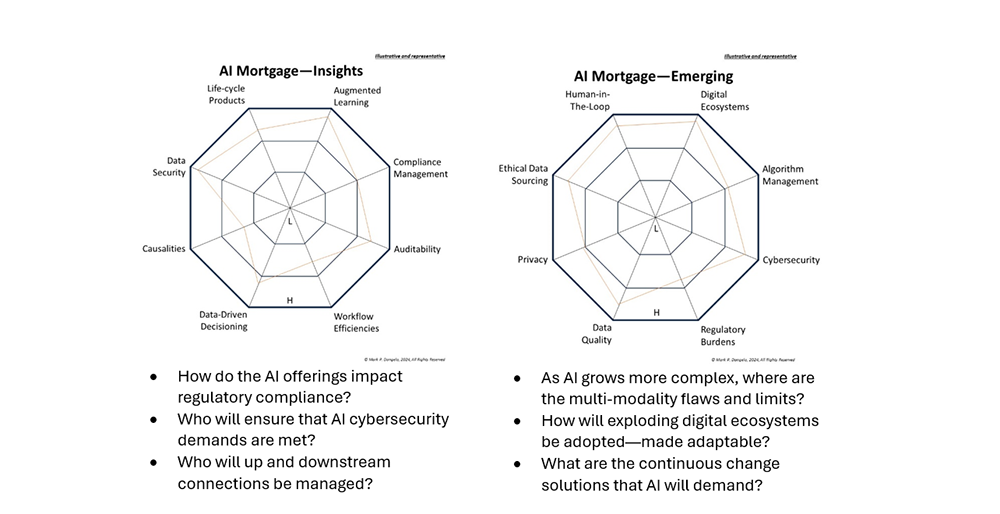
The above diagrams in this section when overlaid against the ones prior project a landscape rooted in strategy and implemented by technology. However, the missing segment that deep experience workforces provide—the missing element of hypergrowth AI—resides in the projection of radar coverage possible when leveraging existing solutions against future domain demands.
AI Legacy Hangover Mitigated Using Experienced Workforces
In the hands of leaders seeking to quantitively leverage experienced workforces using layered AI capabilities, the assembly of the radar charts provide the rationale and implications needed to create a culture, operating model, and profitability that is enabled by AI solutions—not replaced by them.
The figures below provide a next level iteration of the prior charts expanding coverage and usage within small, medium, and large organizations. When applying the features and functions of emerging AI across the traditional and futures, BFSI and mortgage leaders can execute against a roadmap of progression that compliments workforces ensuring greater transparency and accountability.
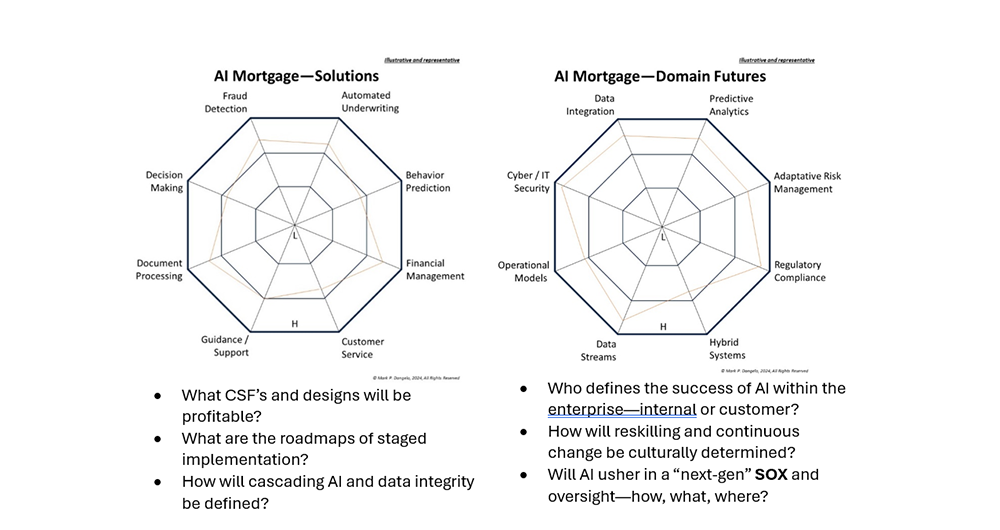
Finally, and implicitly, all AI solutions purchased, tailored and implemented should have a warning label—”results may vary.” Most organizations start with the last two radar charts in this section—skipping the section’s prior baselines, analysis, and gaps illustrated in the prior four.
AI expectations are stratospheric driven by the unprecedented and seemingly unbounded advancements of the last 24 months. However, the principles of operation may initially favor worker replacement, the longer-term realities for AI will reside with experienced domain workforces being the catalyst for sustainable results.
It is worth remembering that what have traditionally experienced as hardware and software legacy cycles were measured in years, decades, and generations. AI solutions will likely be measured in months. Therefore, there will be two foundational pillars that enterprise leaders and industry influencers will have at their disposal and reuse—data (as a product, i.e., DaaP) and experienced personnel.
Given these likely axioms, do we really subscribe to the current generational belief and management theory that AI will eliminate our dependence on aging workforces? Or will those leaders who define the roadmap for AI experienced designs reinvent the industry and customer behaviors?
It is likely that both strategic guidelines will find efficacy, but iterative, exploding AI capabilities in the hands of experienced domain workers will be the greatest opportunity for the next phase of digital reinvention. The untapped value for industry pathfinders and system architects resides not only in efficiencies and insights, but its robust amalgamation with deep domain knowledge workers who are skilled in cascading complexities.
As our workforces age, it is the opportunities at the other end of the spectrum which will deliver sustainable differentiation for BFSI and mortgage industries.
(Views expressed in this article do not necessarily reflect policies of the Mortgage Bankers Association, nor do they connote an MBA endorsement of a specific company, product or service. MBA NewsLink welcomes your submissions. Inquiries can be sent to Editor Michael Tucker or Editorial Manager Anneliese Mahoney.)