
Vinny Souza of Enact Mortgage Insurance; Going Beyond Data-Driven: How Model Outputs are Future Drivers of Decision-Making
Vinny Souza is Head of Data Science with Enact, Raleigh, N.C., formerly Genworth Mortgage Insurance, where he is responsible for revolutionizing enterprise operations with AI and data science solutions. The statements provided are the opinions of Vinny Souza and do not reflect the views of Enact or its management.
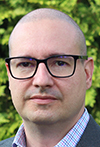
Today, I would venture to say that nearly every organization is well advanced on its mission to better leverage data to make business decisions. In this digital age, businesses have access to an abundance of information that can help them. The trick is putting them to use effectively.
While being data-driven is an important milestone for many, it’s time to move forward to the next destination on this digital journey. Let’s talk about making the jump from data-driven decision-making to model-driven decision-making.
What’s the difference?
On this journey, think of data as an old paper map. It has all the information you need: roads, highway distances, etc. However, much like we did before the internet, you must do the work of planning your route based on the data available on the map. With the information you glean, you can then make a data-driven decision about which route you will take.
Now, think of models like GPS. More specifically, think of any navigational app on your smartphone. Give it a starting location and a destination, and it will plan your route, tell you where the traffic is, list any tolls, and give you the fastest and most efficient route possible. It can even give you options based on directness, fuel efficiency, or where you’d like to stop along the way.
That’s the difference between leveraging data and leveraging data models. We make data-driven decisions when we leverage reports, visualizations, interactive dashboards, and descriptive analytics to extract information and understand the current scenario. These tools tell us what occurred, rather than why it occurred.
Data models put the data to work to derive actionable insights from all the information an organization has. Data models go beyond descriptive analytics and can explain why something happened, predict what can happen next, prescribe the best course of action, and, ultimately, become a “decision optimization engine,” providing us with the best possible path given the operating constraints and indicating the optimal output subject to the environment or scenario programmed into the model.
Having a large amount of data is an asset, but constructing machine learning models to augment the company’s decision-making capabilities is the next key milestone in the digital transformation journey.
Why change?
Ultimately, organizations want to use data to efficiently retain clients, increase employee engagement, expand sales coverage, and maximize profitability through better decision-making.
Rather than spending time merely making sense of the data – month after month, iteration after iteration – companies should increasingly rely on artificial intelligence (AI) and machine learning to do the analysis and enhance actionable insights.
Machine learning models can make predictions and recommendations based on the data used to train the models. As a result, leaders can go beyond observing trends and reacting to elementary insights. These models help inform leaders on the best course of action so they can make better-informed decisions much quicker and spend that time focusing on more complex problems, instead.
The impact of AI and machine learning is important for all industries, but especially for finance functions like mortgage lending. Data models can tell you which market segments to prioritize, how to maximize the efficiency of operations, how to minimize delinquencies, how to create the most effective portfolio mix, and much more.
It might feel more daunting to trust a model than it is to trust just data, but the benefits, like the ones listed above, are significant.
What do model-driven organizations gain?
The first and most obvious benefit is better decision-making. AI and machine learning can look at huge amounts of data – more than any person or team could handle all at once. The resulting decisions are informed by what’s going on in the business, and what’s happening in the market, while also considering happenings in the near and far past or scenario simulations. Data models give organizations an incredibly well-rounded view of their situation and, in turn, help make the best decisions given all the factors affecting the business.
Model-driven organizations also reap efficiency gains. Since machine learning models can be automated and process significant amounts of data at once, it frees staff up to do more complex work. Repetitive tasks and simple operational and process decisions can be eliminated so that staff has more time to spend problem-solving, innovating, and supporting customers or consumers.
AI often carries a negative connotation, such as the fear of job losses, but the goal of these models, however, is not to cut the worker out, but to free them up. AI helps people work smarter, not harder.
Moving Forward
Leveraging data models in decision-making is a key step of any digital transformation effort. However, digital transformation is a journey – an evolution – and every business will use models differently. Regardless of how businesses integrate their model findings, leaders and decision-makers will find that model-driven insights augment their intelligence and push their business forward.
(Views expressed in this article do not necessarily reflect policy of the Mortgage Bankers Association, nor do they connote an MBA endorsement of a specific company, product or service. MBA NewsLink welcomes your submissions. Inquiries can be sent to Mike Sorohan, editor, at msorohan@mba.org; or Michael Tucker, editorial manager, at mtucker@mba.org.)