
Dave Parker of LoanLogics on ‘Confidence Scoring’ and Human Workflow
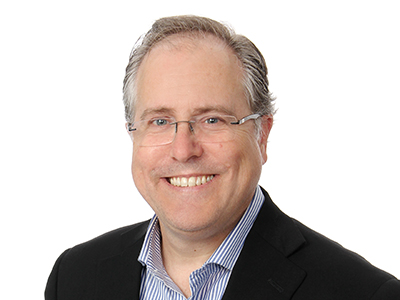
Dave Parker is Chief Product Officer for LoanLogics, a Jacksonville, Fla.-based provider of loan quality technology for mortgage manufacturing and loan acquisition. He is responsible for defining and executing the vision and direction of the company’s product portfolio and designing new solutions for the industry’s current challenges. He has more than 30 years of experience in mortgages and technology. Inquiries can be sent to LoanLogicsInfo@LoanLogics.com.
LoanLogics recently published a white paper, “The Double Act of Human and Machine: a Relationship Story,” available at https://loanlogics.com/wp-doubleact.html?utm_campaign=PR.
MBA NEWSLINK: Can you explain “confidence scoring?” How does it help lower lender costs and optimize human workflow?
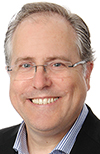
DAVE PARKER, LOANLOGICS: Confidence scoring is about working smarter, not harder. When used with document recognition technology, confidence scoring measures the probability that the results from these technologies are correct. It is not a measure of accuracy. For example, if 100 documents are run through ADR (automated document recognition) and only 13 documents are not recognized correctly, that’s 87% accuracy.
Confidence scores, on the other hand, measure how likely the result for each one of those documents were correct. The 13 documents that weren’t classified correctly probably would typically have lower confidence scores. Using confidence scoring, a company can strategically set scoring thresholds on documents to either pass or trigger a manual review if the confidence score is not high enough, particularly based on how critical the documents are. In the long run, it creates greater efficiencies in mortgage processing and operations as a whole.
NEWSLINK: What are some examples that illustrate how the machine can work alongside human workflow to address industry priorities, such as ROI and profit margins?
PARKER: Let’s say, for example, in the best-case scenario, the cost of human processing is $1 per document and 20 cents per document when processed through technology. Now let’s take 30 documents—10 of those documents are highly critical and the rest are moderately critical. Normally it would take $30 to manually review all those documents. But instead of doing that, we’ll manually review the 10 most critical documents and apply ADR and a confidence score threshold of 90% and above to the 20 other documents.
Now let’s say out of the 20 other documents, 5 fail with a confidence score that is less than 90% and therefore require a manual review, but the rest pass and are allowed to move forward. So instead of spending $30 to manually review every document, we’ve only spent $21 – $6 to run the 30 documents through ADR, $10 to also manually review the critical documents, and $5 to address the five documents that failed. We’ve just cut our human reviews in half and cut our costs by 30%.
NEWSLINK: How do machines improve loan quality?
PARKER: Let’s start at the beginning from the point-of-sale. Today, documents can be sent online or through portals, but believe it or not, many lenders still process these documents manually. Instead, access to real time tools that can classify documents and extract needed data from them can eliminate bottlenecks and streamline workflow. Taking it one step further, some lenders are using API (application programming interface) integrations built between their point-of-sale system, their LOS (loan origination system) and their document management system, which push and pull data where it needs to go, using humans for exception management only, and processing files with little error and greater speed.
Machines can also provide quality oversight for the production process. Loan quality audit reviews using rules-based automation can enable lenders to scale their pre-closing reviews, which is particularly important in a purchase market that typically comes with higher defect rates. Post-closing reviews, whether done in-house or by an audit services provider, can also be optimized by technology that runs credit, collateral, and compliance tests to surface defects, provides an audit trail for human exception management and condition clearing, and includes real-time reporting transparency for monthly projects.
From a cost factor, there’s no need for a company to hire new staff if the current staff uses machines to scale the capacity to manage and improve loan quality.
NEWSLINK: How can other tasks, such as data validation and data formatting, benefit from machine automation?
PARKER: Machine automation can be used to identify whether data extracted from one document is consistent with data from other documents and data in a lender’s LOS. For example, the index rate or margin within the note can be automatically checked to see if it matches the same data in the LOS. Auditors can be immediately alerted of discrepancies and manually review them quickly.
Machine automation can also help transform data fields and formats for exporting files to another application that expects certain values and formats. One example that has traditionally been a challenge is reporting HMDA data. With automated rules, lenders can easily transform the reportable data fields in their loan files to meet the specific formatting requirements laid out by the CFPB for their Loan Application Register submissions. The result, smoother, faster more accurate HMDA reporting.
NEWSLINK: What role do machines play in the secondary mortgage market? For example, how can they improve buying and selling of loans and trading mortgage servicing rights?
PARKER: The biggest challenge in trading MSRs is lack of consistency. There are no standards when it comes to transferring loans, and poor loan delivery leads to longer purchase cycle times, which can also impact pricing. Today, this problem can be solved through a portal using an SFTP (Secure File Transfer Protocol) for bulk loan delivery and APIs for system-to-system connections. This enables buyers to transform loan files from any seller into a configurable, standardized package of data, document naming and stacking order.
NEWSLINK: Lenders will continue to focus on regulatory risks into 2022 and beyond, including TRID and HMDA compliance, and there will likely be new compliance risks. How can machines help to reduce current and new regulatory risks in the next year?
PARKER: Look at what machines are already doing and I think you’ll find your answer. Today, machine automation is capable of performing nearly all of the auditing work for HMDA reviews. Today, auditors using technology are able to complete as many seven to eight post-close loan file reviews per day. Meanwhile, lenders are using compliance and loan quality technology integrations to perform 18 or more compliance reviews per staff member each day. There’s nothing stopping lenders from applying similar combinations of human and machine to solve future regulatory challenges, which are sure to come.
NEWSLINK: How do mortgage lenders prepare internally to integrate machines into their processes? Do you have any examples of the human relationship with the machine inside a mortgage operation?
PARKER: We’ve boiled down technology implementation into several basic steps. First, you have to understand the specific problem you have, how technology will help solve it, and what processes will need to be updated so your team can adapt to the new technology. Second, you need to plan to get data from one system to another. Third, you should establish a timeline and assemble a team to make sure you have the right people involved in the implementation process. Finally, you should be prepared for the chance that some of your early assumptions were incorrect and be willing to change course if need be. In other words, be flexible.
NEWSLINK: What is the industry doing now and what do you plan to see it doing down the road to help drive further applications of machine automation?
PARKER: There is growing industry support for the collection and display of information digitally that opens new possibilities for more automation and greater value for the industry as a whole. Ultimately, as the industry and organizations such as MISMO continue to push to digitize the entire mortgage process, there will be more standardization of data, which will drive further adoption of machine technology.
Next year, I expect more organizations will be prioritizing automation and data and document accuracy, both during production and through the sale or servicing of loan assets. I’m also anticipating new advancements in big data analytics and artificial intelligence, which can help lenders improve profitability while reducing risk throughout the industry in general. As these things happen, we’ll see more human-machine relationships produce even greater efficiencies throughout the mortgage cycle, which will benefit borrowers as well. It’s going to be an exciting ride.
(Views expressed in this article do not necessarily reflect policy of the Mortgage Bankers Association, nor do they connote an MBA endorsement of a specific company, product or service. MBA NewsLink welcomes your submissions. Inquiries can be sent to Mike Sorohan, editor, at msorohan@mba.org; or Michael Tucker, editorial manager, at mtucker@mba.org.)