
Shawn Ansley of VICE Capital Markets: The Need for Strong Data/System Integrations in Hedging
Shawn Ansley is managing director with mortgage hedge advisory firm Vice Capital Markets. Reach him at sansley@vicecapitalmarkets.com.
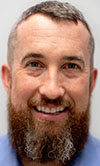
While “The Law of Averages” may apply to a wide variety of activities, hedging isn’t one of them. For a mortgage lender to extract the most value out of their hedging strategy, that strategy must be specific to their organization and its unique make-up. After all, investor relationships, product focus, market goals and risk appetite all play a role in formulating an optimal hedging strategy. What one lender does in any one of these areas is going to differ from what a competitor down the street is doing. If a lender uses someone else’s averages to form its hedging strategy their results may fall short of what is ultimately possible.
Structuring a lender’s specific hedging strategy requires both solid integrations and data inputs to formulate accurate pull-through models and achieve real-time position management. Understanding the role data integrity and integrations play in optimizing a hedging strategy is crucial to maximizing success and the level of profitability that strategy ultimately delivers.
As one would expect, the primary data source needed to form a hedging strategy is a lender’s loan origination system (LOS). While certain platforms dominate the LOS market, not every lender uses the same system, and not every LOS is designed the same way. Each system has its nuances, including the naming conventions for data fields.
When the hedging platform needs a “close date” (i.e. the date when a loan has closed, funded and is eligible for sale), the LOS may use “funded date” to denote this same information. Lenders will need to do a certain amount of data mapping between their LOS and hedging platform to ensure the right data is being pulled from the LOS and populated in the correct corresponding field within the hedging platform to ensure accuracy. For example, many lenders have a data field for an expected close date. If data from this field is used rather than the final close date field, the hedge model would indicate a 100% pull-through rate, which is inaccurate and would ultimately inhibit a lender’s ability to manage their position effectively.
Of course, the specific data points lenders need to pull will vary based on their execution strategy and investor relationships. While there are standard data points that lenders will need to pull every single time (lock date, expiration, closed date, purchase dates, loan purpose and more), each investor has its own requirements on what information they want to be included within a data file for loans to be priced for purchase.
This becomes especially critical when executing bulk sales, as bid tapes need to contain all of the data points required by every bidder. Some examples of unique data points bidders have requested include the number of borrowers on the loan, the borrower’s first name or whether the loan featured a property inspection waiver. As time is always of the essence for these transactions, knowing what bidders expect to see ahead of time and ensuring those points are included in the data pull from the LOS prevents costly delays in putting loans out for pricing.
Lenders must also be aware of the impact certain activities have on the data being used to formulate their hedging strategy, particularly in regards to their fallout and pull-through analyses. Locked loans, closed loans and loans with terminal statuses form the triad of the fallout analysis, which determines a lender’s expected level of rate exposure and ultimately helps maintain an optimal hedge to cover that risk.
Some LOS platforms remove all lock information for loans that get canceled, which impacts the fall-out analysis. If this data is missing, it then appears as if that loan was never locked to begin with, never hedged and, therefore, cannot count as fallout – all of which is not the case. Thus, lenders need to be mindful of these instances and institute failsafe or backup measures to ensure this critical information is retained in some capacity.
Ultimately, practicing good data hygiene goes a long way in building a solid foundation for a hedging strategy. Weeding out test loans before they make their way into the pipeline, confirming that all required data fields have been completed and double-checking data entries to ensure the information has been entered into the correct data field can all significantly improve the quality of the data upon which the hedging strategy rests.
Given the dynamic nature of today’s mortgage environment, lenders can ill afford to rely on generalities or averages to maximize their secondary execution and profitability. A truly effective hedging strategy is based on sound and accurate data proprietary to the lender for whom it is being developed. Otherwise, lenders may find themselves falling victim to Murphy’s Law rather than the Law of Averages.
(Views expressed in this article do not necessarily reflect policy of the Mortgage Bankers Association, nor do they connote an MBA endorsement of a specific company, product or service. MBA NewsLink welcomes your submissions. Inquiries can be sent to Mike Sorohan, editor, at msorohan@mba.org; or Michael Tucker, editorial manager, at mtucker@mba.org.)