
Mark Dangelo: Establishing a Foundation for AI Growth and Profitability—Part Two
Mark P. Dangelo is Chief Innovation Consultant with BlackFin Group, Laguna Hills, Calif., responsible for leading and managing innovation-led business transformation and technology projects and innovation-based advisory services. He is also president of MPD Organizations LLC and an adjunct professor of graduate studies in innovation and entrepreneurship at John Carroll University. He is the author of four innovation books and numerous articles and a regular contributor to MBA NewsLink. He can be reached at mark@mpdangelo.com or at 440/725-9402.
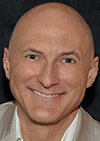
When financial services and banking organizational (#FSBO) leaders talk about innovation, there is one topic that receives not only the top response, but a panacea prospect, a carnal allure, and an anticipatory large financial bet for differentiation—I am talking about artificial intelligence (#AI).
Whereas some of you may envision humanoid robots, others jump to “thinking machines” capable of replacing repetitive tasks thereby allowing humans to leverage the machines working in an orchestrated score to exceed customer expectations and anticipate future actions and behaviors. Or perhaps it is just a version of HAL, the Terminator, the Matrix, Transformers, “I, Robot”, or HumaGears (beware humankind).
Regardless of the imaginary, these expectations of the advancement of an early-stage, emerging set of technologies are influencing markets that are poised from a shift of volume to one defined by consolidation and likely searches for efficiencies. Is there a crisis dealing with efficiencies? AI is the answer! Is there a skills problem? AI is the new employee. Is there a demand for complex predictions for customer offerings? Then AI is the synthetic intelligence that can deliver flawlessly. However, how will FSBOs implement AI? Can it be leveraged? Do we have the capability to consistently deliver?
To reinforce this urgency across FSBO executive ranks, the statistics of intent and current deployments show a vast push to implement AI—but is the organization ready given the poor returns already experienced? The following statistical diagram sets the stage for 2021-2025.
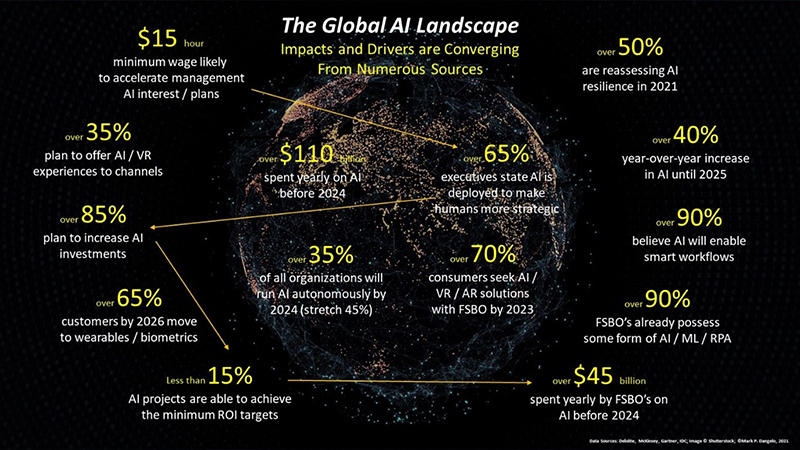
Moving into tomorrow, the acceptance of AI is a given. However, the value and sustainability of AI—the other part of the evolving story—few teams explore beyond their software vendor’s promises (now being pushed as part of the “next competitive advantage”). However, being a contrarian, if all your competitors are deploying it and they have the same (vendor) software as you, then how are these AI offerings a non-commodity or customer differentiator? Perhaps before the arrival of this Fourth Industrial Revolution (also called digital ecosystems, circular economies, et al), the plans of attack for market relevance adhered to the IT philosophies of using single focused innovational advancements, while adhering to a familiar playbook. AI is not going to play by traditional IT implementation ideals. AI is not a single dimensional innovation.
Indeed, as I noted in a prior month’s article (Mark P. Dangelo: Establishing a Foundation for AI Growth and Profitability – MBA Newslink), the arrival of AI signifies a change in the definition, development, and implementation of these accelerating, multi-faceted intelligent learning solution sets. AI demands new definitional predictors and success measures. It demands new independent verification and validation (IV&V) testing harness. It demands new compartmentalized containers that can be assembled in layers, reassembled in new layers, and retired at a container level to accommodate AI advances that are exhibiting a four times greater advancement cycle than the widely panned Moore’s Law.
As we examine the state of emerging AI solutions and expectations, there must be a place to begin as we watch the progression of software and hardware accelerate to a crescendo by 2025. Indeed, and by using a picture as a thousand words, we can see below that we must start with a “tell, involve, teach, learn” AI lifecycle. Within the lifecycle, business impacts and AI differentiations branch out into definitional demands and operating constructs that then can be evaluated against financial and competitive factors.
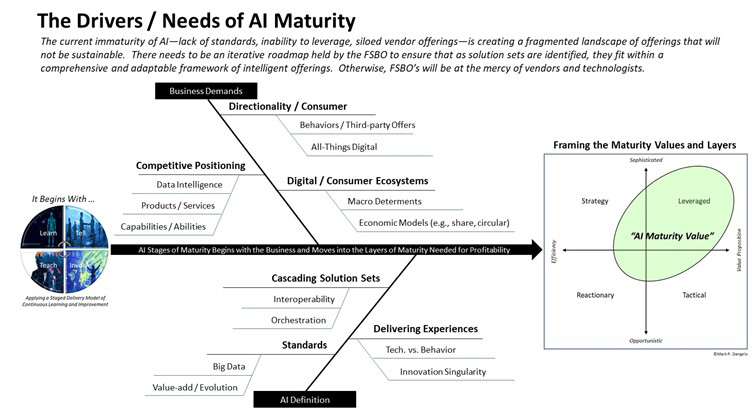
Yet, the demarcations do not end there. As the diagram above indicates, the creeping definition and commitment of AI starts not with the technology or vendor offering—but much farther up the supply chain. To reach an “AI Maturity Value” FSBO leadership must thinking differently and approach the use of AI systems as business not ordinary.
AI is not a one-off still common within the implementation of IT systems. The factors that must be present before successful AI can be leverage for a FSBO resides with the inability to think holistically, while implementing in compartments and layers. The inability to compartmentalize AI will, as functionality increases and as the interconnectedness expands, depress anticipated returns. Moreover, the ability to implement new emerging AI capabilities will, if prior solution sets are not deployed with operational and intelligence boundaries, create systems that will be consistently error prone and unable to find a “fit” within the FSBO supply chains.
As FSBOs we find ourselves an innovation tip of spear moment. AI is progressing rapidly. Our competitors (or so it seems) are deploying AI solutions. Our organization is preparing for Capex AI implementations to provide efficiencies and advanced predictive capabilities. However, before we drink the “AI Kool-Aid” are we ready for realities once the prototype has completed and the pilot projects are underway? Let us touch on a few of the dozens of lessons learned gleaned from those who have previously struggled with the realities of AI in its infancy.
- What is the strategy and architecture to “glue” AI together within our FSBO industry and our own solution sets to ensure that the building blocks chosen fit with current and future offerings?
- What will be the impacts to skills, processes, and partners to our operating model and value propositions when AI impacts our business models and value propositions?
- As IT moves from traditional into AI non-traditional testing and implementation, how will this impact our internal culture and who will lead us?
- How will FSBOs react when AI goes astray and the algorithmic webs of capabilities that have created differentiated competitive advantages are no longer meeting success factors with rising “intelligence” abilities? Stated differently, what happens when AI is outside its limits?
- As AI webs grow more complex and capable, can they be trusted not just internally, but by consumers, regulators, investors, and socially accepted norms?
- With AI possessing deep learning capacity due to unchecked data gathering spurred by digital strategies, what will the cybersecurity landscape become and what will the lines of defense be by the end of 2021?
- How will regulators enact compliance guidelines for learning technology that is increasingly beyond the control of predictability due to the progression of algorithmic webs (i.e., regulations are written to address knowns—not unknowns)?
- When algorithmic collisions happen (and they will with greater frequency with wider AI solution sets), what is the conflict resolution process?
- With the typical FSBO having hundreds to thousands of APIs deployed, how will the movement towards AI replace these ETL, feeds, and augmentation snippets?
Indeed, there are dozens more questions, depending on your unique market position and strategic vision that need to be asked—and answered, and then readdressed due to the hyper-advancements with the broad field of what is labeled AI. It is very doubtful that a single prescriptive approach of AI will work, but AI frameworks of definition, development, and implementation if adopted properly and guided using experienced teams will create sustainability and adaptability on the AI journey over the next decade.
Moving this idea of a framework for AI success forward I have created a model to channel AI ideas and the targets of solutions into pragmatic layers of how to define and mature AI for FSBOs.
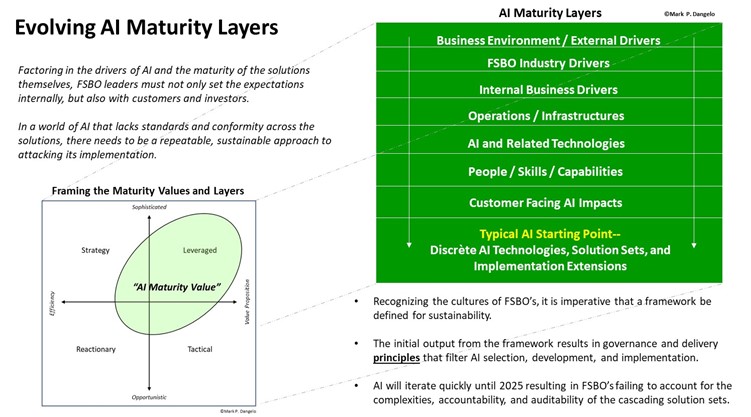
As I have ascertained, AI is embryonic in its capabilities. The promise of solution set capability is unprecedented, yet the preconditions necessary for not only initial prototyping but sustainable adaptation, are foreign to all but the largest of FSBO’s. AI requires, in fact it demands, an ability to leverage vast quantities of non-contaminated data (both internally and externally) to be able to learn across its algorithms. AI does not represent an incremental addition to an IT solution—it is a comprehensively new approach that leverages humans, it does not replace them.
Now if we investigate the composites of these conceptual layers, we can see vast opportunities and strengths with AI that leverages their highly skilled human counterparts. By decomposing the layers of
AI into discrete building blocks, the progression of unique, competitively differentiated AI offerings can be demonstrated (see below Innovation A, B or C). By using a layering approach rather than merely adopting an AI solution will yield consistent and long-term profitability and efficiency.
A Starting Point– Breaking AI into Composite, Building Block Layers
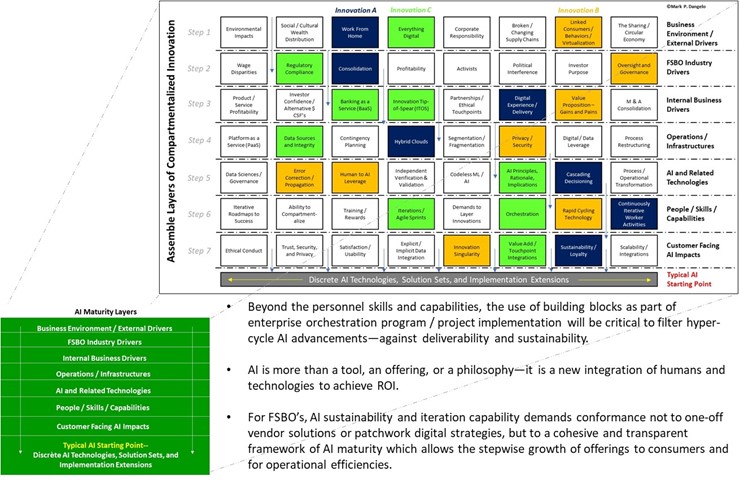
The last three years have been a sea change for the possibilities of AI from its humble beginnings as a computer science discipline of neural networks. As we now can see, using the framework above, the starting point for AI success is not the implementation of a one-off vendor solution. While the insight from deploying a point-based prototype offering can be valuable to assess skills, culture, and operational challenges, the sustainable use of AI across the enterprise will, if not addressed using the maturity model above, likely lead to a less than 15% ROI success rate (see initial diagram).
For IT personnel, the use of container and assembly of building blocks is not a foreign concept. For business individuals, this layered approach to AI innovation allows for smart investing, targeted opportunity leverage, and the leadership to create action plans that are iterative and compartmentalized (thereby avoiding potentially large project failures and significant write offs).
Today, if we hit the reset button and start down a stepwise journey with AI, the FSBO organization can apply proven principles (e.g., JIT) to these intelligent solutions without jeopardizing the brand, its customers, and its profitability. If after reading this article, the concepts and implementation frameworks for the future still seem opaque, you are not alone. AI is rooted in mathematical, data, and computer science that is cutting edge.
AI represents today rudimentary thinking machines—tomorrow they will represent layers of business-driven technological layers that are assembled and reassembled in cascading solutions which leverage human intelligence—not as a comprehensive replacement. AI is just moving forward from the decades of computer scientists (like me) trying to find a means to move procedural systems into autonomous assistants. The book is being written—the story has just begun. FSBOs need to create their own roadmaps and be comfortable with emerging solutions. An AI maturity framework of interconnected layers and compartments is a good composition to build future success.
(Views expressed in this article do not necessarily reflect policy of the Mortgage Bankers Association, nor do they connote an MBA endorsement of a specific company, product or service. MBA NewsLink welcomes your submissions. Inquiries can be sent to Mike Sorohan, editor, at msorohan@mba.org; or Michael Tucker, editorial manager, at mtucker@mba.org.)